Risk prediction models for hepatocellular carcinoma in different populations
Introduction
Liver cancer is one of the most aggressive malignant neoplasms worldwide, which ranks the 5th and 9th most common cancer among males and females, respectively, and the incidence of liver cancer is the highest in East and South-East Asia, Northern and Western Africa (1). An estimated 782,000 new cases and 745,000 deaths occurred worldwide during 2012 (2). During the last few decades, the incidence rate of liver cancer has increased in most developed countries (3).
The disease burden of hepatocellular carcinoma (HCC) is high and no effective treatments are available so far (4). But possible interventions, such as interferon therapy for chronic hepatitis B (CHB), chronic hepatitis C (CHC) or lifestyle changes are available to reduce the mortality or alter the course of the disease, on condition that individuals at high risk can be identified. Therefore, simple, easily administered, and applicable risk prediction models of HCC would be of great value in terms of public health view. Recently, several risk prediction models of HCC in different populations have been presented with simple, efficient, and ready-to-use parameters. In this review, the features of these prediction models were summarized, and perspectives of these prediction models were also discussed.
Risk factors of HCC
Approximately 85% of the HCC cases occur in developing countries every year, and the highest incidence rates are described in regions where hepatitis B virus (HBV) infection is endemic: Southeast Asia and sub-Saharan Africa (5, 6, 7,8). Globally, over 350 million people are chronically infected with HBV (9), and HBV accounts for 53% of total HCC cases and virtually all childhood HCC cases (10, 11).
Hepatitis C virus (HCV) infection predominates in Europe, North America, Japan, Pakistan, Mongolia, and Egypt (12). According to the statistics of World health Organization (WHO), about 3% of the world’s population has been infected with HCV and 170 million are chronic carriers. Prevention of HCC associated with HCV infection is currently one of the most important issues in public health worldwide.
Besides HBV or HCV carriers, HCC also occurs in noncarriers of these viruses (13, 14). This may be attributable to other potential risk factors, such as dietary aflatoxin exposure, alcohol consumption, smoking, obesity, diabetes, chronic liver diseases. In some western countries where HCC incidence is low, heavy alcohol consumption, obesity and diabetes mellitus (DM) played important roles in liver carcinogenesis development (15). In South China and sub-Saharan Africa, digestions of dietary aflatoxin could partly contribute to the risk of HCC (16). Approximately one-eighth of all HCC cases in Japan are unrelated to HBV or HCV infection (17, 18). Lifestyle or chronic disease-related factors such as alcohol consumption, obesity and diabetes are considered to be independently associated with the risk of HCC (19, 20, 21, 22, 23, 24, 25). Some additional established risk factors of HCC include iron overload, family history of malignant liver tumors, and possibly tobacco exposure (26, 27).
Risk prediction models of HCC in CHB patients
Chronic carriers of HBV have an increased risk of HCC ranges from 5-fold to 98-fold and with a population attributable risk of 8% to 94% (28). It is very important to identify high-risk patients among carriers of CHB. This will have important implications for treatment allocation and strategic screening of HCC.
Characteristics of study populations for model derivation
By October 2015, several risk prediction models of HCC were developed in CHB cohorts, of which, three studies named the GAG-HCC score (29), CU-HCC score (30) and LSM-HCC score (31) were from Hong Kong, China. The GAG-HCC score was derived from a cohort in Liver Clinic, Queen Mary Hospital, University of Hong Kong. Patients who had received or were receiving any form of established antiviral treatment for CHB were excluded and their follow-up time was censored at the time of initiation of treatment. The CU-HCC score and LSM-HCC score were developed from the CHB cohorts referred to Prince of Wales Hospital of Chinese University of Hong Kong.
Another four studies named REACH-B score (32), REACH-B nomogram (33), Lin-HCC score (34) and LeeHCC score (35) were all derived from the cohorts consisted of CHB patients from the R.E.V.E.A.L.-HBV Study in Taiwan, China. Briefly, they were community-based cohorts of participants seropositive for hepatitis B surface antigen (HBsAg) and seronegative for antibodies against hepatitis C virus (antiHCV). The participants did not have cirrhosis at study entry and did not receive antiviral treatment during follow-up. The last one was named Kim-HCC score (36) which was built from a cohort of patients with CHB who visited the outpatient clinic and received a transient elastography examination in the Institute of Gastroenterology, Korea.
In clinical practice, the GAG-score was the first model for risk prediction in evaluating the risk of HCC in CHB patients with the integration of possible independent factors. The REACH-B nomogram recruited the largest cohort of 3,653 patients so far to develop the risk model which was predictive of HCC incidence in CHB patients with the longest period of follow-up for about 12 years. Selection bias in the development cohorts in Taiwan was minimized because the cohorts of CHB patients were taken from a population-based cohort. Table 1 shows the cohorts’ characteristics of risk prediction models in CHB patients.

Full table
Characteristics of risk prediction models
Most risk prediction models of HCC in CHB patients included the following factors: age, gender, HBV-DNA (37), hepatitis B e antigen (HBeAg) (38) and alanine aminotransferase (ALT) (39). Considering that gender differences were usually in HBV-related HCC, even if gender was not statistically significant in the CU-score, LSM-score and Lin-score, the Lin-score was still including gender in its three risk models with an assigned score of 1. It was the first risk model that incorporates liver-related seromarkers such as alpha-fetoprotein (AFP) (40), serum aspartate transaminase (AST)/ALT ratio (AAR) (41), gamma-glutamyltransferase (GGT) (42) and alpha-1 globulin (43) to predict the risk of HCC. It is demonstrated that most of the predictors included in models were also seromarkers for cirrhosis and both risk models (model Ⅱ and model Ⅲ) of the Lin-score, which developed from the REACH-B score (model Ⅰ), had good predictability for HCC risk, regardless of cirrhosis status.
Among the studies which included the patients having cirrhosis at entry, cirrhosis/liver stiffness measure (LSM) was an indispensable factor (44, 45, 46). The LSM-score refined the CU-HCC score with LSM as the component replacing clinical cirrhosis. This new LSM-HCC score including LSM as a factor was superior in terms of accuracy and sensitivity to predict the risk of HCC than the CU-HCC score which classified cirrhosis only into two categories (yes or no).
As a factor, the point of quantitative serum HBsAg levels was noteworthy in the Lee-HCC score. The level of serum HBsAg was assessed to be an independent risk factor of HCC development as well as a response assessment to HCC surveillance and antiviral therapy. The Lee-HCC score was the first time simultaneously developing the prediction models to predict the risk of both long-term cirrhosis and HCC risk. But the models of risk factors were different in predicting cirrhosis and HCC. Supplementary Table S1 shows the characteristics of risk prediction models in CHB patients.

Full table
External validation and discrimination
Most of these risk prediction models were lack of external validation to a satisfactory extent except the REACH-B score. The REACH-B score had a validation on a cohort of 1,505 patients from three hospitals in South Korea and Hong Kong, China. In the overall validation analyses, the model showed fairly good discrimination capabilities, with the area under receiver operating curve (AUROC) equal to 0.80 [95% confidence interval (95% CI), 0.78-0.82] for 5-year prediction, and 0.77 (95% CI, 0.75-0.79) for 10-year prediction, respectively. It did not show the sensitivity and specificity for cutoff score to detect HCC, while plotting ROC curves for 3-year, 5-year, and 10-year risks with the 1,505 patients from the validation cohort. They also plotted a calibration chart for predicted and observed risks. The overall model showed a fairly good discrimination capability, with AUROC of 0.81 (95% CI 0.79-0.83) for risk at 3 years, 0.80 (0.78-0.82) at 5 years, and 0.77 (0.75-0.79) at 10 years. A calibration chart with both predicted and observed risks of HCC, showing good correlation in the validation cohort. The correlation coefficients between observed risk of HCC and mean predicted risk estimated by risk prediction model were greater than 0.9, showing excellent calibration characteristics of the risk prediction tool.
Limitations
The following are some common limitations in these risk prediction models. First, in the cohorts of Korea and Hong Kong, China, the patients recruited in hospital-based studies were more likely to have active disease. So the rate of HCC in training cohort is higher than that in patients with CHB infection only. However, more patients with chronic HBV would belong to the low-risk category in the primary care setting, and this would further increase the negative predictive value of the model for external validation.
Second, in the studies, all patients were from East Asians and risk factors for HCC may be different in other ethnic groups where fewer patients acquire HBV perinatally. The models needed to be independently validated before generalization to China Mainland, Northern and Western Africa and Southern Europe. It might also need to take into account diversities in age at infection (perinatal vs. adulthood), genetic background, HBV genotype or species and exposure to environmental factors such as dietary aflatoxin and alcohol intake.
Third, the seromarkers in these risk prediction models may be dynamic and the changing patterns should be associated with clinical liver outcomes. Incorporating not only the baseline values of seromarkers, but also the follow-up values or changes which may increase the predictabilities of risk models and make them more reliable and accurate.
Last but not least, antiviral therapy created a confounding effect on the performance of the risk prediction model (47). But the patients in these HCC score studies did not receive antiviral therapy except the CU-HCC, LSM-HCC and Kim-HCC scores. The previous two models had the antiviral therapy rates of 15.1% and 38.0%, respectively, in the derivation cohort, and 25.0% and 32.0%, respectively, in the validation cohorts. The Kim-HCC score having no validation cohort had the antiviral therapy rate of 37.8% in the whole cohort.
Risk prediction models of HCC in cirrhosis, CHC patients and general population
Most available risk prediction models for HCC were limited to individuals at elevated risk who were carrying HBV. There were small numbers of HCC risk prediction models for cirrhosis and CHC patients. And it was useful to estimate risk based not only on cirrhosis or hepatitis virus infection status, but also on prevalent and modifiable lifestyle-related factors. So simple and easy-to-administer risk prediction model which is based on commonly available data in general population would be also of great value. In a word, risk prediction models of HCC in cirrhosis, CHC patients and general population provided useful guides for people in high and average risk.
Characteristics of study populations for model derivation
By October 2015, two risk prediction models termed ADRESSHCC score (48) and AFP-Based Algorithm (49) which were used for patients with cirrhosis were developed. The ADRESSHCC score based on a large U.S. cohort with cirrhosis of various etiologies and severe liver disease waitlisted for liver transplantation at 127 U.S. liver transplant centers. The AFPBased Algorithm identified 11,721 patients with HCV related cirrhosis from national Department of Veterans Affairs Clinical Case Registry, in whom 35,494 AFP tests were performed. A predictive model that included data on levels of AFP, ALT, and platelets, along with age at time of AFP test, best discriminated between patients who did and did not develop HCC.
Two risk prediction models for patients with CHC came from the cohorts of patients who were from Chang Gung Memorial hospital of Taiwan, China. The first one was the Chang-HCC score (50) predicting the risk of HCC from patients who received combined pegylated interferon (PEGIFN) and ribavirin therapy to achieve sustained virological response (SVR). The second model was the Chang-HCC score (51) derived from a cohort of patients who were treated with interferon-based therapies.
In general population, three predictive scoring systems for HCC had been introduced. The first one was the MichikawaHCC score (52) derived from the Japan Public Health Centerbased Prospective Study Cohort II, which covered a Japanese general population who participated in health checkups, including HBsAg and anti-HCV measurements. In Japan, screening for HBV and HCV is carried out during regular health checkups (4), so individuals can obtain information on their hepatitis virus infection status without further medical consultation. The Michikawa-HCC score also developed an additional model for subjects who were positive of anti-HCV and negative of HBsAg. The second one was the Wen-HCC score (53) which was established from a prospective cohort from a standard medical screening program conducted by the MJ Health Management Institution in Taiwan, China. Because tests for HCV infection were performed at an extra cost to members in Taiwan, only a subset of participants has the data on HCV status. So the studies divided the cohort into two subcohorts in order to provide more accurate risk prediction estimates: one cohort had the HCV test, and the other did not. The last model was the Hung-HCC score (54) which analyzed a large number (n=12,377) of subjects from three different observational cohorts (55, 56, 57, 58) of Taiwan, China, heavily enriched in subjects with HBV infection. There were differences in the cohort compositions of gender, HBsAg status, and HCC family history because of the cohort recruitment policy for special interests.
It could be found that the ADRESS-HCC score and AFPBased Algorithm were the only two models derived from the U.S. cohorts, making applicability to calculate the annual incidence of HCC in North American population. The two Chang-HCC models were the only prediction models of HCC for patients who received antiviral therapy such as interferon, PEG-IFN and ribavirin combination therapy. Currently, the antiviral therapy can reduce HCC development and prolong survival in patients. So people with hepatitis virus infection were usually treated with antiviral therapy. However, patients who were able to achieve antiviral therapy remained at risk of developing HCC. Both of the two risk prediction models of HCC in general population had much larger sample size of subjects than risk prediction models in high-risk patients (Michikawa-HCC score: 17,654 subjects, Wen-HCC score: 428,584 subjects). And the subjects in the Michikawa-HCC score and Wen-HCC score were from community-based cohorts, which help to minimize the selection bias. Table 1 also shows the characteristics of the cohorts of risk prediction models in cirrhosis, CHC patients and general population.
Characteristics of risk prediction models
Age and gender were still common factors in these risk prediction models. Compared with other prediction models, risk prediction models in patients with cirrhosis included some unique factors such as race (non-Hispanic white vs. other), etiology of cirrhosis (viral vs. metabolic/alcohol vs. autoimmune groups) and the child-turcotte-pugh (CTP) score. The CTP score was calculated for each individual at listing based on the individual values for total bilirubin, international normalized ratio, and albumin in addition to the documentation of ascites (none, slight, or moderate) and hepatic encephalopathy (none, grade 1/2, or grade 2/3) as previously described (59).
SVR was a special factor in the risk prediction models of HCC in CHC patients. Patients with HCV infection were treated with IFN, PEG-IFN and ribavirin therapy, and achieved SVR (60, 61, 62). In the Chang-HCC score (SVR), all patients were achieving SVR while in the Chang-HCC score, SVR was used as a prediction factor for HCC. Even though patients were able to achieve a SVR, they remained at risk of developing HCC (63, 64). SVR reduced the risk of HCC as it was showed in the Chang-HCC score. If patients can achieve SVR, the average 5-year risk reduction of HCC was 4.8%, and the 10-year risk reduction was 10.7% when taking into account the effects of other factors.
An important output is the net benefit of surveillance, a weighted difference between true- and false-positive classifications. The Hung-HCC score was the only model using a decision curve analysis (DCA) to determine the level of risk that was associated with benefit to surveillance. The model was superior to the current age-based criteria for subjects when this was plotted against the threshold probability for developing HCC.
To our knowledge, detailed clinical data could be readily available for the high-risk individuals but much less available for those at average or unknown risk. Besides the factors included in prediction models for high-risk population, body mass index (BMI), physical activity, diabetes, smoking, alcohol consumption, coffee consumption, and other environmental factors should be considered in the risk prediction models for average-risk subjects in different populations. Supplementary Table S2 shows the characteristics of risk prediction models in cirrhosis, CHC patients and general population.
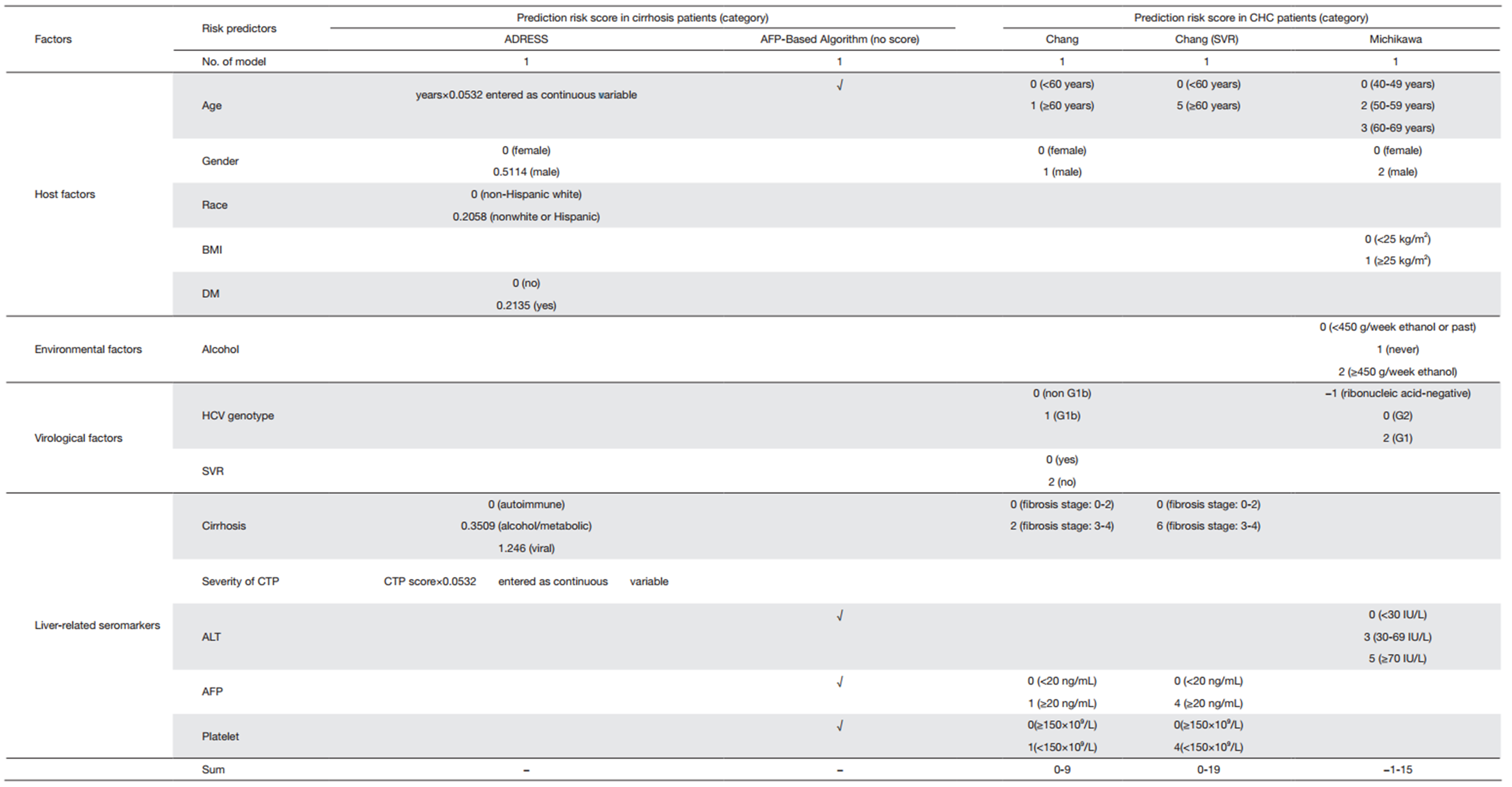
Full table
External validation and discrimination
Of the 5 risk prediction models of HCC in cirrhosis, CHC patients and general population, only the ADRESS-HCC score had the external validation to examine the performance of the model using patients with cirrhosis enrolled in the HALT-C cohort (62). Of 1,050 HCV patients enrolled in the HALT-C study, 426 had cirrhosis at the time of enrollment and were included in the validation analysis. Patients with other concomitant liver diseases or evidence of hepatic decompensation were excluded. The C-index, a measure of discrimination (an assessment of how well the model is able to separate individuals into those who will and will not develop HCC) (65), of the ADRESS-HCC score was 0.69 (95% CI, 0.67-0.71). When comparing the low-risk group with the intermediate-risk and high-risk groups (ADRESS-HCC score of 4.71), the prediction model was found to have such a high sensitivity of 0.93. Calibration chart comparing predicted and observed risks of HCC was also plotted, and good correlation in validation cohort was observed in the chart. Some additional good performances of the model for patients with prevalent, incident HCC, or those without HCC increased our confidence in the applicability of the ADRESS-HCC score to individuals with varying severity of liver dysfunction. Moreover, the ADRESS-HCC score is appropriate to the cirrhosis patients in the U.S. who were potentially eligible for transplant and other populations whose etiology of liver disease was similar to that in the ADRESS-HCC model.
Limitations
The limitations of risk prediction models in cirrhosis, CHC patients and general population are partly similar to those in CHB patients which mentioned previously. Some additional limitations were presented as below.
First, the validation cohort for HCC risk prediction in CHC patients only included cirrhosis patients with confirmed HCV infection so the performance of model in patients with liver disease from other possible etiologies could not be examined.
In addition, whether the patients had ever received antiviral therapy was not mentioned in detail for AFP-based Algorithm and ADRESS-HCC score, which enrolled the subjects with cirrhosis. Based on clinical practice guidelines (66, 67), subjects with compensated cirrhosis should receive antiviral therapy in the absence of contraindications. If these patients received antiviral therapy, both risk prediction models based on serum AFP levels, ALT or the severity of CTP could be biased (68, 69). While the subjects in these studies, which derive the risk prediction models in CHC patients, were all received antiviral treatment. So there was a lack of standard guidance about the assessment of HCC risk in CHC patients without antiviral therapy.
Lastly, it had no information about the treatment of the participants with HBV and/or HCV infection received before or during the study period in the prediction models of HCC in general population. Since the possible effects of treatment were not considered in the development of the two prediction models, the risk estimations should be interpreted with caution.
Current difficulties and future directions in HCC risk prediction models
Additional molecular markers improving prediction
These risk prediction models above included mainly routine clinical indexes because they are more likely to be used in reallife clinical practice and were already available in the study cohorts. But there are additional HCC risk factors, which are not commonly considered in those scores, for example, HBsAg level, HBV genotype, host mutations, HBV mutations, antiHCV and platelet.
HCC is genetically a heterogeneous cancer. Few gene polymorphisms conferred more than a 2-fold increase in HCC risk (70, 71, 72). It is unlikely that a single mutation will have sufficient impact on HCC prediction. While for HBV mutations, besides the GAG-score using it as a predict factor, the study by Yin et al. reported that combo mutations with C1653T, T1753V and A1762T/G1764A observably improved the validity of HCC prediction by age, male and cirrhosis (73).
These molecular markers may be useful in clinical practice when they have become more widely available and are better evaluated in combination with traditional risk factors.
Interracial external validation
Different risk prediction models had different characteristics of cohorts and predicting factors. Even they had partly the same predicting factor, the weighting of risk factors was slightly different. So some concerns are needed to ensure that the score used most closely matches the population from which the patient comes. For example, in North America for patients with advanced liver disease, the ADRESS-HCC score and AFP-Based Algorithm are most likely to be the most accurate, whereas one of the Asian-derived scores is more likely to be appropriate for hepatitis B in Asians.
Nowadays the challenge is obvious whether risk prediction models can be applied to other ethnic groups and gradually build up the risk prediction models with more stability and applicability or not. Except for the REACH-B and ADRESSHCC scores, the other HCC predictive models have been either validated with samples drawn from the same cohort as the derivation sets, limited by a lack of stringent validation for the applicability to other population, or even have no internal validation.
Only 2 having-external-validation models, the REACH-B and ADRESS-HCC scores, had similar ethnic group or characteristics validation to the derivation sets. Therefore, interracial external validation taking into account diversities in age at infection, genetic background, virus genotype or species, etc., is urgently needed for HCC prediction models, because the most rigorous test of a risk prediction score is applying it to other populations with different characteristics.
Optimize HCC risk predictions for patients on antiviral therapy
Antiviral therapy is the common treatment in patients at the risk of HCC. Based on clinical practice guidelines, subjects with compensated cirrhosis should receive antiviral therapy in the absence of contraindications (66, 67). If these patients received antiviral therapy, most virological risk factors and ALT, AFP, cirrhosis may alter (68, 69, 74). It is unclear how to interpret changes in risk scores after antiviral therapy.
Most risk prediction models in CHB patients were constructed in untreated patients. The performance of HCC risk scores in treated patients has been tested in a cohort of 1,531 patients from Hong Kong, China (75). Although the study suggested that the HCC risk models can also be applied in treated patients to predict future HCC development, the risk is considerably lower than that of untreated patients and it should be noted that not all components of the risk scores were important HCC risk factors. In particular, a baseline or on-treatment HBV DNA level of ≥2,000 IU/mL was no longer associated with the incidence of HCC (75). This was consistent with a recent validation in the era of antiviral therapy, which modified REACH-B score where LSM values were incorporated into REACH-B score instead of serum HBV-DNA levels, having the better prognostic performances for HCC development at 3-/5-year in patients with antiviral therapy (n=848), compared to other prediction models (CUHCC, GAG-HCC, REACH-B, and LSM-HCC scores). However, in patients without antiviral therapy (n=460), it had the predictive performances comparable to those of other models (76).
Accurate diagnosis of cirrhosis
Current treatment guidelines recommended liver biopsy in patients with borderline treatment indications (67, 77, 78), but the procedure was limited by its poor patient acceptance and invasiveness. In routine clinical practice, the diagnosis of cirrhosis is commonly based on abdominal ultrasonography or clinical characteristics of portal hypertension. Early cirrhosis is often undiagnosed and the risk of HCC would be underestimated. The necessity of using ultrasonography as a surveillance tool for HCC is not altered in many models and accuracy of these models is greatly limited.
Recently, LSM using transient elastography has been introduced as a promising noninvasive method for assessing the degree of liver fibrosis, with considerable accuracy and high reproducibility (79, 80). It is highly reproducible and has been validated against liver histology in patients with CHB (44, 81, 82). Because LSM can accurately be used for the diagnosis of cirrhosis, it comes as no surprise that patients with high LSM have increased risk of HCC. Besides transient elastography, acoustic radiation force impulse, shear-wave elsatography and magnetic resonance elastography also provide physical measurements of liver elasticity or stiffness and have been used for the diagnosis of cirrhosis (83, 84, 85). However, these new techniques have not been widely assessed. The optimal cut-offs and their roles in HCC prediction are yet to be defined.
Conclusions
Over 10 risk prediction models of HCC across different populations were constructed so far. Risk prediction models can be used to predict the risk of subsequent HCC development in different populations such as CHB, cirrhosis, CHC and general population. No scoring system is going to have 100% predictability. All the available risk scores performed well, although some were better than others. Generalized authorization of risk prediction models should be confirmed using internal and external validation with a prospective manner in different populations. These simple tools accurately assess HCC risk among different groups with average or high risk. It may be helpful for further pursuing public health and clinical approaches in reducing the risk of HCC.
Risk prediction models have not yet been widely used in medical and health services, because they were not from homogeneous characteristics of the study. Moreover, all of them were constructed from different numbers of sample size, different median times of follow-up, different areas and different ways of validation as well. Simple, efficient and accurate prediction models of HCC development constructed from readily available variables on clinical, laboratory, community settings are necessary for performing strategic processes, such as screening, surveillance, diagnosis and treatment of HCC in different populations. It is expected that standardized consensus on HCC risk prediction models, combining host factors, environmental factors, virological factors and liver-related seromarkers, which is based on the simple, veracious and assessable characteristics and available in real clinical, laboratory and community settings, should be established. Moreover, generalized authorization of risk prediction models needs to be confirmed by internal and external validations designed by prospective method in different populations of regions with epidemiologic versatility of HCC.
Acknowledgements
We would like to thank the original studies for their contribution to this review.
Funding: This work was supported by funds from the National Key Basic Research Program “973 project” (2015CB554000), the State Key Project Specialized for Infectious Diseases of China (No. 2008ZX10002-015 and 2012ZX10002008-002), and the Foundation for Innovative Research Groups of the National Natural Science Foundation of China (Grant No. 81421001).
Footnote
Conflicts of Interest: The authors have no conflicts of interest to declare.
References
- Torre LA, Bray F, Siegel RL, et al. Global cancer statistics, 2012. CA Cancer J Clin 2015;65:87-108. [PubMed]
- Ferlay J, Soerjomataram I, Dikshit R, et al. Cancer incidence and mortality worldwide: sources, methods and major patterns in GLOBOCAN 2012. Int J Cancer 2015;136:E359-86. [PubMed]
- Salhab M, Canelo R. An overview of evidence-based management of hepatocellular carcinoma: a meta-analysis. J Cancer Res Ther 2011;7:463-75. [PubMed]
- Tsukuma H, Tanaka H, Ajiki W, et al. Liver cancer and its prevention. Asian Pac J Cancer Prev 2005;6:244-50. [PubMed]
- Nishioka K, Levin AG, Simons MJ. Hepatitis B antigen, antigen subtypes, and hepatitis B antibody in normal subjects and patients with liver disease. Bull World Health Organ 1975;52:293-300. [PubMed]
- Prince AM, Szmuness W, Michon J, et al. A case/control study of the association between primary liver cancer and hepatitis B infection in Senegal. Int J Cancer 1975;16:376-83. [PubMed]
- Trichopoulos D, Tabor E, Gerety RJ, et al. Hepatitis B and primary hepatocellular carcinoma in a European population. Lancet 1978;2:1217-9. [PubMed]
- Wong VW, Chan HL. Prevention of hepatocellular carcinoma: a concise review of contemporary issues. Ann Hepatol 2012;11:284-93. [PubMed]
- Lavanchy D. Hepatitis B virus epidemiology, disease burden, treatment, and current and emerging prevention and control measures. J Viral Hepat 2004;11:97-107. [PubMed]
- Perz JF, Armstrong GL, Farrington LA, et al. The contributions of hepatitis B virus and hepatitis C virus infections to cirrhosis and primary liver cancer worldwide. J Hepatol 2006;45:529-38. [PubMed]
- El-Serag HB. Epidemiology of viral hepatitis and hepatocellular carcinoma. Gastroenterology 2012;142: 1264-73. [PubMed]
- Raza SA, Clifford GM, Franceschi S. Worldwide variation in the relative importance of hepatitis B and hepatitis C viruses in hepatocellular carcinoma: a systematic review. Br J Cancer 2007;96:1127-34. [PubMed]
- Blonski W, Kotlyar DS, Forde KA. Non-viral causes of hepatocellular carcinoma. World J Gastroenterol 2010;16:3603-15. [PubMed]
- Chen JG, Zhang SW. Liver cancer epidemic in China: past, present and future. Semin Cancer Biol 2011;21:59-69. [PubMed]
- Hassan MM, Hwang LY, Hatten CJ, et al. Risk factors for hepatocellular carcinoma: synergism of alcohol with viral hepatitis and diabetes mellitus. Hepatology 2002;36:1206-13. [PubMed]
- IARC Working Group on the Evaluation of Carcinogenic Risks to Humans. Some traditional herbal medicines, some mycotoxins, naphthalene and styrene. IARC Monogr Eval Carcinog Risks Hum 2002;82:1-556. [PubMed]
- Tanaka H, Imai Y, Hiramatsu N, et al. Declining incidence of hepatocellular carcinoma in Osaka, Japan, from 1990 to 2003. Ann Intern Med 2008;148:820-6. [PubMed]
- Umemura T, Ichijo T, Yoshizawa K, et al. Epidemiology of hepatocellular carcinoma in Japan. J Gastroenterol 2009;44 Suppl 19:102-7. [PubMed]
- Inoue M, Iwasaki M, Otani T, et al. Diabetes mellitus and the risk of cancer: results from a large-scale population-based cohort study in Japan. Arch Intern Med 2006;166:1871-7. [PubMed]
- Inoue M, Kurahashi N, Iwasaki M, et al. Metabolic factors and subsequent risk of hepatocellular carcinoma by hepatitis virus infection status: a large-scale population-based cohort study of Japanese men and women (JPHC Study Cohort Ⅱ). Cancer Causes Control 2009;20:741-50. [PubMed]
- Ohishi W, Fujiwara S, Cologne JB, et al. Risk factors for hepatocellular carcinoma in a Japanese population: a nested case-control study. Cancer Epidemiol Biomarkers Prev 2008;17:846-54. [PubMed]
- Ohki T, Tateishi R, Sato T, et al. Obesity is an independent risk factor for hepatocellular carcinoma development in chronic hepatitis C patients. Clin Gastroenterol Hepatol 2008;6:459-64. [PubMed]
- Tanaka K, Tsuji I, Wakai K, et al. Alcohol drinking and liver cancer risk: an evaluation based on a systematic review of epidemiologic evidence among the Japanese population. Jpn J Clin Oncol 2008;38:816-38. [PubMed]
- Yang WS, Va P, Bray F, et al. The role of pre-existing diabetes mellitus on hepatocellular carcinoma occurrence and prognosis: a meta-analysis of prospective cohort studies. PLoS One 2011;6:e27326. [PubMed]
- Yang WS, Shu XO, Gao J, et al. Prospective evaluation of type 2 diabetes mellitus on the risk of primary liver cancer in Chinese men and women. Ann Oncol 2013;24:1679-85. [PubMed]
- Gao J, Xie L, Yang WS, et al. Risk factors of hepatocellular carcinoma--current status and perspectives. Asian Pac J Cancer Prev 2012;13:743-52. [PubMed]
- Yang Y, Wu QJ, Xie L, et al. Prospective cohort studies of association between family history of liver cancer and risk of liver cancer. Int J Cancer 2014;135:1605-14. [PubMed]
- Chen CJ, Yu MW, Liaw YF. Epidemiological characteristics and risk factors of hepatocellular carcinoma. J Gastroenterol Hepatol 1997;12:S294-308. [PubMed]
- Yuen MF, Tanaka Y, Fong DY, et al. Independent risk factors and predictive score for the development of hepatocellular carcinoma in chronic hepatitis B. J Hepatol 2009;50:80-8. [PubMed]
- Wong VW, Chan SL, Mo F, et al. Clinical scoring system to predict hepatocellular carcinoma in chronic hepatitis B carriers. J Clin Oncol 2010;28:1660-5. [PubMed]
- Wong GL, Chan HL, Wong CK, et al. Liver stiffness-based optimization of hepatocellular carcinoma risk score in patients with chronic hepatitis B. J Hepatol 2014;60:339-45. [PubMed]
- Yang HI, Yuen MF, Chan HL, et al. Risk estimation for hepatocellular carcinoma in chronic hepatitis B (REACH-B): development and validation of a predictive score. Lancet Oncol 2011;12:568-74. [PubMed]
- Yang HI, Sherman M, Su J, et al. Nomograms for risk of hepatocellular carcinoma in patients with chronic hepatitis B virus infection. J Clin Oncol 2010;28:2437-44. [PubMed]
- Lin YJ, Lee MH, Yang HI, et al. Predictability of liverrelated seromarkers for the risk of hepatocellular carcinoma in chronic hepatitis B patients. PLoS One 2013;8:e61448. [PubMed]
- Lee MH, Yang HI, Liu J, et al. Prediction models of longterm cirrhosis and hepatocellular carcinoma risk in chronic hepatitis B patients: risk scores integrating host and virus profiles. Hepatology 2013;58:546-54. [PubMed]
- Kim do Y, Song KJ, Kim SU, et al. Transient elastographybased risk estimation of hepatitis B virus-related occurrence of hepatocellular carcinoma: development and validation of a predictive model. Onco Targets Ther 2013;6:1463-9. [PubMed]
- Chen CJ, Yang HI, Su J, et al. Risk of hepatocellular carcinoma across a biological gradient of serum hepatitis B virus DNA level. JAMA 2006;295:65-73. [PubMed]
- Yang HI, Lu SN, Liaw YF, et al. Hepatitis B e antigen and the risk of hepatocellular carcinoma. N Engl J Med 2002;347:168-74. [PubMed]
- Kim HC, Nam CM, Jee SH, et al. Normal serum aminotransferase concentration and risk of mortality from liver diseases: prospective cohort study. BMJ 2004;328:983. [PubMed]
- Durazo FA, Blatt LM, Corey WG, et al. Des-gammacarboxyprothrombin, alpha-fetoprotein and AFP-L3 in patients with chronic hepatitis, cirrhosis and hepatocellular carcinoma. J Gastroenterol Hepatol 2008;23:1541-8. [PubMed]
- Giannini E, Risso D, Botta F, et al. Validity and clinical utility of the aspartate aminotransferase-alanine aminotransferase ratio in assessing disease severity and prognosis in patients with hepatitis C virus-related chronic liver disease. Arch Intern Med 2003;163:218-24. [PubMed]
- Sawabu N, Nakagen M, Ozaki K, et al. Clinical evaluation of specific gamma-GTP isoenzyme in patients with hepatocellular carcinoma. Cancer 1983;51:327-31. [PubMed]
- Eriksson S, Carlson J, Velez R. Risk of cirrhosis andprimary liver cancer in alpha 1-antitrypsin deficiency. N Engl J Med 1986;314:736-9. [PubMed]
- Wong GL, Wong VW, Choi PC, et al. Assessment of fibrosis by transient elastography compared with liver biopsy and morphometry in chronic liver diseases. Clin Gastroenterol Hepatol 2008;6:1027-35. [PubMed]
- Wong VW, Wong GL, Chan FK. Liver stiffness measurement: simplicity is prerequisite for reliability. Gastroenterology 2013;144:243-4. [PubMed]
- Jung KS, Kim SU, Ahn SH, et al. Risk assessment of hepatitis B virus-related hepatocellular carcinoma development using liver stiffness measurement (FibroScan). Hepatology 2011;53:885-94. [PubMed]
- Liaw YF, Chu CM. Hepatitis B virus infection. Lancet 2009;373:582-92. [PubMed]
- Flemming JA, Yang JD, Vittinghoff E, et al. Risk prediction of hepatocellular carcinoma in patients with cirrhosis: The ADRESS-HCC risk model. Cancer 2014;120:3485-93. [PubMed]
- El-Serag HB, Kanwal F, Davila JA, et al. A new laboratorybased algorithm to predict development of hepatocellular carcinoma in patients with hepatitis C and cirrhosis. Gastroenterology 2014;146:1249-55. [PubMed]
- Chang KC, Hung CH, Lu SN, et al. A novel predictive score for hepatocellular carcinoma development in patients with chronic hepatitis C after sustained response to pegylated interferon and ribavirin combination therapy. J Antimicrob Chemother 2012;67:2766-72. [PubMed]
- Chang KC, Wu YY, Hung CH, et al. Clinical-guide risk prediction of hepatocellular carcinoma development in chronic hepatitis C patients after interferon-based therapy. Br J Cancer 2013;109:2481-8. [PubMed]
- Michikawa T, Inoue M, Sawada N, et al. Development of a prediction model for 10-year risk of hepatocellular carcinoma in middle-aged Japanese: the Japan Public Health Center-based Prospective Study Cohort Ⅱ. Prev Med 2012;55:137-43. [PubMed]
- Wen CP, Lin J, Yang YC, et al. Hepatocellular carcinoma risk prediction model for the general population: the predictive power of transaminases. J Natl Cancer Inst 2012;104:1599-611. [PubMed]
- Hung YC, Lin CL, Liu CJ, et al. Development of risk scoring system for stratifying population for hepatocellular carcinoma screening. Hepatology 2015;61:1934-44. [PubMed]
- Huang HH, Shih WL, Li YH, et al. Hepatitis B viraemia: its heritability and association with common genetic variation in the interferon gamma signalling pathway. Gut 2011;60:99-107. [PubMed]
- Yu MW, Chang HC, Chen PJ, et al. Increased risk for hepatitis B-related liver cirrhosis in relatives of patients with hepatocellular carcinoma in northern Taiwan. Int J Epidemiol 2002;31:1008-15. [PubMed]
- Yu MW, Chang HC, Liaw YF, et al. Familial risk of hepatocellular carcinoma among chronic hepatitis B carriers and their relatives. J Natl Cancer Inst 2000;92:1159-64. [PubMed]
- Yu MW, Shih WL, Lin CL, et al. Body-mass index and progression of hepatitis B: a population-based cohort study in men. J Clin Oncol 2008;26:5576-82. [PubMed]
- Pugh RN, Murray-Lyon IM, Dawson JL, et al. Transection of the oesophagus for bleeding oesophageal varices. Br J Surg 1973;60:646-9. [PubMed]
- Manns MP, McHutchison JG, Gordon SC, et al. Peginterferon alfa-2b plus ribavirin compared with interferon alfa-2b plus ribavirin for initial treatment of chronic hepatitis C: a randomised trial. Lancet 2001;358:958-65. [PubMed]
- Fried MW, Shiffman ML, Reddy KR, et al. Peginterferon alfa-2a plus ribavirin for chronic hepatitis C virus infection. N Engl J Med 2002;347:975-82. [PubMed]
- Di Bisceglie AM, Shiffman ML, Everson GT, et al. Prolonged therapy of advanced chronic hepatitis C with low-dose peginterferon. N Engl J Med 2008;359:2429-41. [PubMed]
- Yoshida H, Tateishi R, Arakawa Y, et al. Benefit of interferon therapy in hepatocellular carcinoma prevention for individual patients with chronic hepatitis C. Gut 2004;53:425-30. [PubMed]
- Poynard T, Marcellin P, Lee SS, et al. Randomised trial of interferon alpha2b plus ribavirin for 48 weeks or for 24 weeks versus interferon alpha2b plus placebo for 48 weeks for treatment of chronic infection with hepatitis C virus. International Hepatitis Interventional Therapy Group (IHIT). Lancet 1998;352:1426-32. [PubMed]
- Harrell FE Jr, Lee KL, Mark DB. Multivariable prognostic models: issues in developing models, evaluating assumptions and adequacy, and measuring and reducing errors. Stat Med 1996;15:361-87. [PubMed]
- European Association for Study of Liver. EASL Clinical Practice Guidelines: management of hepatitis C virus infection. J Hepatol 2014;60:392-420. [PubMed]
- European Association for Study of Liver. EASL clinical practice guidelines: Management of chronic hepatitis B virus infection. J Hepatol 2012;57:167-85. [PubMed]
- Asahina Y, Tsuchiya K, Nishimura T, et al. α-fetoprotein levels after interferon therapy and risk of hepatocarcinogenesis in chronic hepatitis C. Hepatology 2013;58:1253-62. [PubMed]
- Di Bisceglie AM, Sterling RK, Chung RT, et al. Serum alphafetoprotein levels in patients with advanced hepatitis C: results from the HALT-C Trial. J Hepatol 2005;43:434-41. [PubMed]
- Clifford RJ, Zhang J, Meerzaman DM, et al. Genetic variations at loci involved in the immune response are risk factors for hepatocellular carcinoma. Hepatology 2010;52:2034-43. [PubMed]
- Jiang DK, Sun J, Cao G, et al. Genetic variants in STAT4 and HLA-DQ genes confer risk of hepatitis B virus-related hepatocellular carcinoma. Nat Genet 2013;45:72-5. [PubMed]
- Zhang H, Zhai Y, Hu Z, et al. Genome-wide association study identifies 1p36.22 as a new susceptibility locus for hepatocellular carcinoma in chronic hepatitis B virus carriers. Nat Genet 2010;42:755-8. [PubMed]
- Yin J, Wang J, Pu R, et al. Hepatitis B virus combo mutations improve the prediction and active prophylaxis of hepatocellular carcinoma: a clinic-based cohort study. Cancer Prev Res (Phila) 2015;8:978-88. [PubMed]
- Wong VW, Wong GL, Chim AM, et al. Surrogate end points and long-term outcome in patients with chronic hepatitis B. Clin Gastroenterol Hepatol 2009;7:1113-20. [PubMed]
- Wong GL, Chan HL, Chan HY, et al. Accuracy of risk scores for patients with chronic hepatitis B receiving entecavir treatment. Gastroenterology 2013;144:933-44. [PubMed]
- Jung KS, Kim SU, Song K, et al. Validation of hepatitis B virus-related hepatocellular carcinoma prediction models in the era of antiviral therapy. Hepatology 2015;62:1757-66. [PubMed]
- Liaw YF, Kao JH, Piratvisuth T, et al. Asian-Pacific consensus statement on the management of chronic hepatitis B: a 2012 update. Hepatol Int 2012;6:531-61. [PubMed]
- Lok AS, McMahon BJ. Chronic hepatitis B: update 2009. Hepatology 2009;50:661-2. [PubMed]
- Kim SU, Seo YS, Cheong JY, et al. Factors that affect the diagnostic accuracy of liver fibrosis measurement by Fibroscan in patients with chronic hepatitis B. Aliment Pharmacol Ther 2010;32:498-505. [PubMed]
- Wong GL, Wong VW, Choi PC, et al. Development of a non-invasive algorithm with transient elastography (Fibroscan) and serum test formula for advanced liver fibrosis in chronic hepatitis B. Aliment Pharmacol Ther 2010;31:1095-103. [PubMed]
- Chan HL, Wong GL, Choi PC, et al. Alanine aminotransferase-based algorithms of liver stiffness measurement by transient elastography (Fibroscan) for liver fibrosis in chronic hepatitis B. J Viral Hepat 2009;16:36-44. [PubMed]
- Marcellin P, Ziol M, Bedossa P, et al. Non-invasive assessment of liver fibrosis by stiffness measurement in patients with chronic hepatitis B. Liver Int 2009;29:242-7. [PubMed]
- Leung VY, Shen J, Wong VW, et al. Quantitative elastography of liver fibrosis and spleen stiffness in chronic hepatitis B carriers: comparison of shear-wave elastography and transient elastography with liver biopsy correlation. Radiology 2013;269:910-8. [PubMed]
- Shi Y, Guo Q, Xia F, et al. MR elastography for the assessment of hepatic fibrosis in patients with chronic hepatitis B infection: does histologic necroinflammation influence the measurement of hepatic stiffness? Radiology 2014;273:88-98. [PubMed]
- Zhang D, Chen M, Wang R, et al. Comparison of acoustic radiation force impulse imaging and transient elastography for non-invasive assessment of liver fibrosis in patients with chronic hepatitis B. Ultrasound Med Biol 2015;41:7-14. [PubMed]