Identification of liver metastasis-associated genes in human colon carcinoma by mRNA profiling
Introduction
Colon carcinoma is one of the most common malignant diseases with 945,000 new cases every year and is the fourth cause of cancer-related deaths worldwide (1). Unfortunately, about 70% of colon carcinoma patients develop liver metastases. Curative-intent resections can be performed in only 10%−15% of liver metastases (2). In the majority of metastatic patients, the standard treatment remains palliative chemotherapy. However, most colon cancer patients with active metastasis appear to be resistant, or even non-responsive, to current treatments. A major clinical challenge is to explore possible therapeutic targets that are specifically expressed in liver metastatic settings.
There have been many attempts to determine predictive factors or explain the underlying mechanisms for distant metastasis. MicroRNA 34a, microRNA-34a-5p, microRNA-340 are associated with colon carcinoma cell proliferation and metastasis (3,4). In addition, the CpG island methylator phenotype (CIMP) is concordant between primary colon carcinoma and distant metastases (5). Mitogen-activated protein kinase (MAPK) and phosphatidylinositol 3-kinase (PI3K) signaling pathways inhibit metastasis to the liver (6). Alterations in gene expression, protein expression, posttranslational modification, microRNA and linc-RNA have been reported to act a part of role in tumor progression. However, these have not revealed effective predicted factor which is specific to liver metastasis. Transcriptomic changes inherit from genomic information and take place before protein level. Therefore, we attempt to investigate the malignant features of hepatic metastasis microenvironment by RNA-sequencing.
Gene expression profiling has become a strategy to identify genes involved in the progression and the prognosis of different cancers. Few attentions were focused on the gene signatures associated with metastatic disease (7). Two studies presented gene signatures associated with metastatic disease containing more than 400 genes. Such long lists of genes are difficult to be used for the development of new therapies (8,9). Pairs of primary and metastatic tumors were analyzed and the samples clustered by patients but not the tissue origin (10,11). The identified genes are specific to colon carcinoma and hepatic metastases, but the precise target is still unknown (12). Comparative profiling of primary colon carcinomas and liver metastases identifies lymphoid enhancer factor-1 (LEF1) as a prognostic biomarker (13). However, this research only focused on the development of diagnostic and prognostic markers without trying to identify gene signatures able to distinguish metastatic from primary cancer tissues (13). Therefore, it is most important for us to investigate effective targets for the treatment of liver metastasis.
To identify genes implicated in metastatic colonization of the liver in colon carcinoma, we compared mRNA expression between groups of normal colon mucosa (N), primary tumors (T) and liver metastases (M) samples which from Gene Expression Omnibus (GEO) database. The expression of the differential genes was processed by gene ontology (GO), Kyoto Encyclopedia of Genes and Genomes (KEGG) orthology and Signal network, which are all effective bioinformatics analytical methods. We then verified the clinical significance of identified genes using clinical samples. Our data provide novel information and help further understanding of the liver metastasis cascade of colon carcinoma.
Materials and methods
Microarray data
The transcriptional expression data (GSE49355 and GSE62321) of human colon tumor were downloaded from the GEO database. They were from the same set of patients. It contained 18 normal colon mucosa (N), 20 primary tumors (T) and 19 liver metastases (M) samples. Platforms information were GPL96 [HG-U133A] and GPL97 [HG-U133B] Affymetrix Human Genome U133A/B Array and the datasets were already normalized.
Investigating of differential expression genes (DEGs)
Genes were standardized and interpreted functionally before comparison. Using random variance model (RVM) t-test (14) and the normal colon mucosa group as the control group, the P value and the false discovery rate (FDR) were calculated for each DEG. FDR was calculated to correct the P-value, which controls type I errors. With a threshold of P<0.05, FDR<0.05 and fold change (FC) >2, DEGs were picked out.
Hierarchical cluster analysis
Hierarchical cluster analysis was performed to ensure good characterizations of screened DEGs between different groups (15). In hierarchical cluster analysis, Pearson correlation was used to calculate the correlation between the genes and samples.
Venn analysis
To identify specific genes of liver metastasis, genes expression in each tissue were input to the web tool Venn Diagrams (http://bioinformatics.psb.ugent.be/webtools/Venn).
GO annotation analysis
Functional analysis of differentially expressed genes was carried out by the GO project (http://www.geneontology.org) on the basis of biological process (16).
Pathway annotation analysis
Pathway analysis was used to identify significant pathways involving DEGs, according to KEGG, BioCarta, and Reactome.
Co-expression network analysis
For each pair of genes, the Pearson correlation coefficient was calculated, and 0.8 was defined as the threshold to construct the network. Within the network analysis, degree of the association is an important factor to determine the relative importance of a gene. We have employed different colors and sizes of node to discriminate the degree of the associations for one gene with the surrounding nodes. The co-expression networks were constructed by Cytoscape (17).
PPI network construction
In order to reveal functional associations between proteins in a genome-wide scale, STRING online tool (18,19) was used to construct a PPI network. In the PPI network, each node represents a protein, and each edge represents an interaction of pairwise proteins. The nodes with a relatively large number of edges were defined as hub proteins.
Gene set enrichment analysis (GSEA)
GSEA was performed by the GSEA software and gene sets used in this work were downloaded from the Molecular Signatures Database. The MSigDB collects various types of gene set and the online pathway database included 1,320 Canonical pathways derived from the pathway databases of BioCarta, KEGG, PID, Reactome and others databases. The data for GSEA analysis is from The Cancer Genome Atlas (TCGA).
TCGA database analysis
TGCA database was derived from UCSC Cancer Browser (https://genome-cancer.ucsc.edu). Overall survival (OS) analysis of colon cancer patients with high and low levels of different genes was shown by using a Kaplan-Meier survival plot. The cut-off values for the genes were the median respectively. We used Kaplan-Meier curves to present the prognosis of the high and low groups. The Wilcoxon log-rank test was then conducted on the Kaplan-Meier curves to detect the survival difference between these two groups. All survival analysis was conducted using the R software.
Clinical specimens
Specimens were from colon carcinoma patients who were diagnosed and received operation in the Department of Anus and Intestine Surgery of the First Affiliated Hospital of Zhengzhou University (Zhengzhou, China) from 2011 to 2013. Pre- and post-operative clinical data and other survival-related data were perfected by reviewing the medical records and following-up the patients by telephone. All postoperative specimens were examined by one pathologist and reviewed by another pathologist. Of them, all patients were used as the basis of the present study. The clinical data of the patients are shown in Table 1. Collection of samples in this study was approved by Institutional Ethics Committee of the First Affiliated Hospital of Zhengzhou University (Ethics approval number: Science-2010-LW-1213), and informed consent was obtained from each patient with available follow-up information.
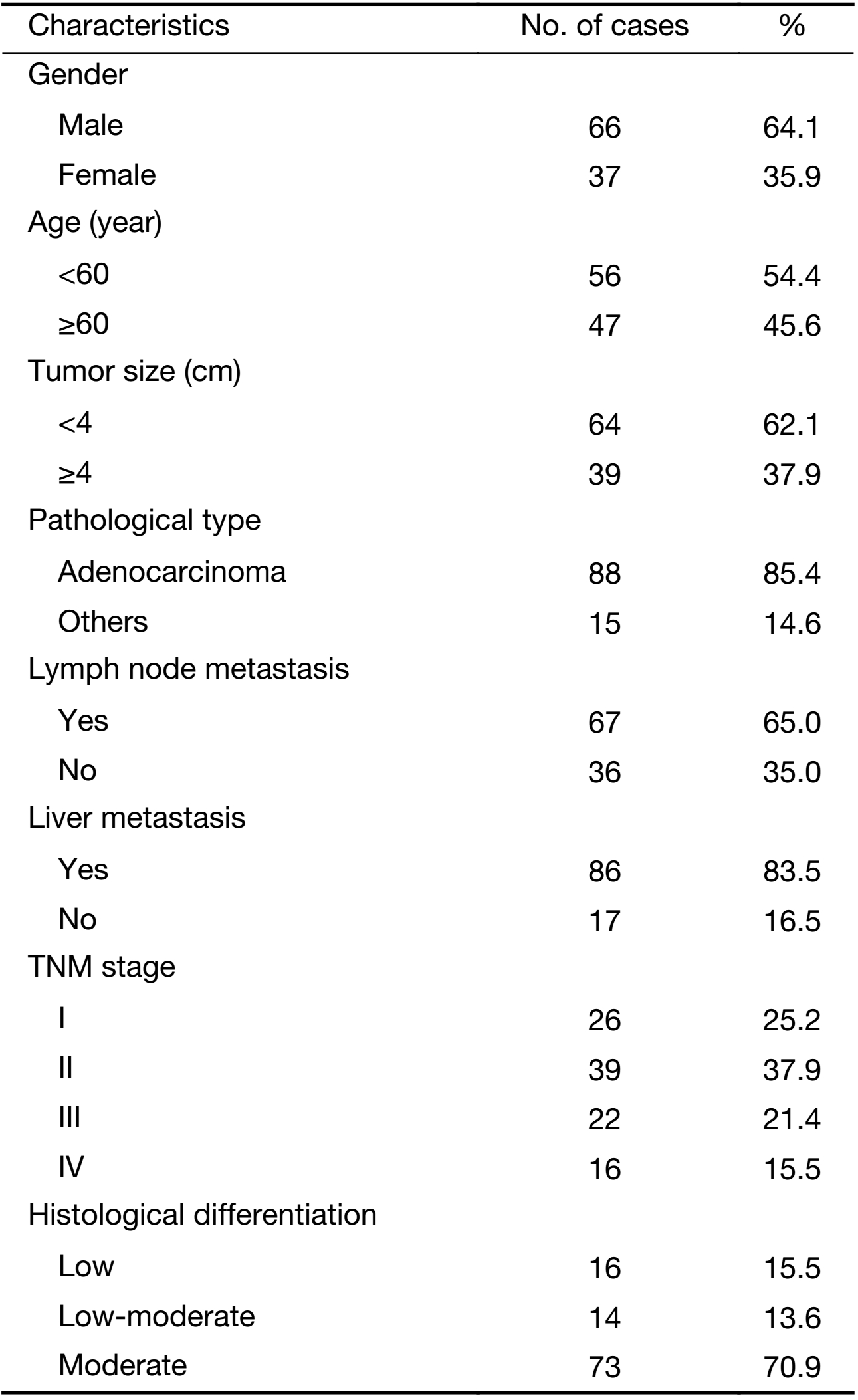
Full table
Quantitative real-time polymerase chain reaction (qRT-PCR)
Tumor or marginal tissues were cut into 20 mm of pieces and mechanically grinded. Then, total RNA was extracted using Trizol solution (Invitrogen, Waltham, MA, USA). qRT-PCR was performed using specific primers and SYBR Green qPCR Master Mix (Takara, Japan). Listed primers were used: 5’-GGAGCCAAAAGGGTCATCATCTC-3’ sense primer and 5’-GAGGGGCCATCCACAGTCTTCT-3’ antisense primer for GAPDH, 5’- CGCTACAGCGACGTGAAGAA-3’ sense primer and 5’-GTTCCAGGCGTTGTACCAC-3’ antisense primer for CXC chemokine ligand 14 (CXCL14). GAPDH was used as an internal control. With the 2-ΔΔCt method, we compared the expression level of clinical samples (20). For each sample, the expression of CXCL14 as well as GAPDH was examined, the relative expression of CXCL14 was calculated by using the 2-ΔCt value of CXCL14 dividing the 2-ΔCt value of GAPDH (20).
Immunohistochemistry
Paraffin-embedded tissues of 45 colon cancer samples were examined for the expression of CXCl14 protein (Abcam, Cambridge, UK; 1:200). Sections were treated with 3% H2O2 and 5% bull serum albumin (BSA) and incubated with primary antibodies overnight at 4 °C. After incubation with horseradish peroxidase (HRP)-conjugated secondary antibody for 1 h at 37 °C, sections were washed and counterstained with hematoxylin, and visualized under a microscope (Olympus, Shinjuku, Japan) (21).
Statistical analysis
Clinicopathologic factors were compared by using the χ2 test and continuous variables were compared by using the Student t test or one-way analysis of variance (ANOVA) analysis. Kaplan-Meier analysis and the log-rank test were used for survival analysis. Univariate and multivariate logistic regression models identified the association between CXCL14 expression and clinical characteristics. P<0.05 was considered statistically difference. All statistics associated with clinical samples were performed using Prism 7 (GraphPad Software Inc., La Jolla, USA). Statistical analysis of significance was calculated by ANOVA followed by Tukey’spost hoc test with SPSS 16.0 for Windows (SPSS Inc., Chicago, IL, USA). The bioinformatics analysis was used by using R software (Version 3.4; R Foundation for Statistical Computing, Vienna, Austria).
Results
Gene expression analysis
We used the public transcriptome sequencing dataset (GSE49355 and GSE62321) from GEO database, including 18 normal colon mucosa (N), 20 primary tumors (T) and 19 liver metastases (M) samples. Detailed sample information could be found in Supplementary Table S1. Expression profiling of the 57 samples was conducted on Affymetrix human U133A/B chips. Expression profiling of the 57 samples was conducted on Affymetrix human U133A chips containing 22,200 probes corresponding to about 12,700 genes. These gene expression data have been performed normalization and log2 transformation. Hierarchical cluster analysis showed that normal samples clustered together and were relatively well separated from T and M samples in GSE49355 and GSE62321 (Figures 1A−D).
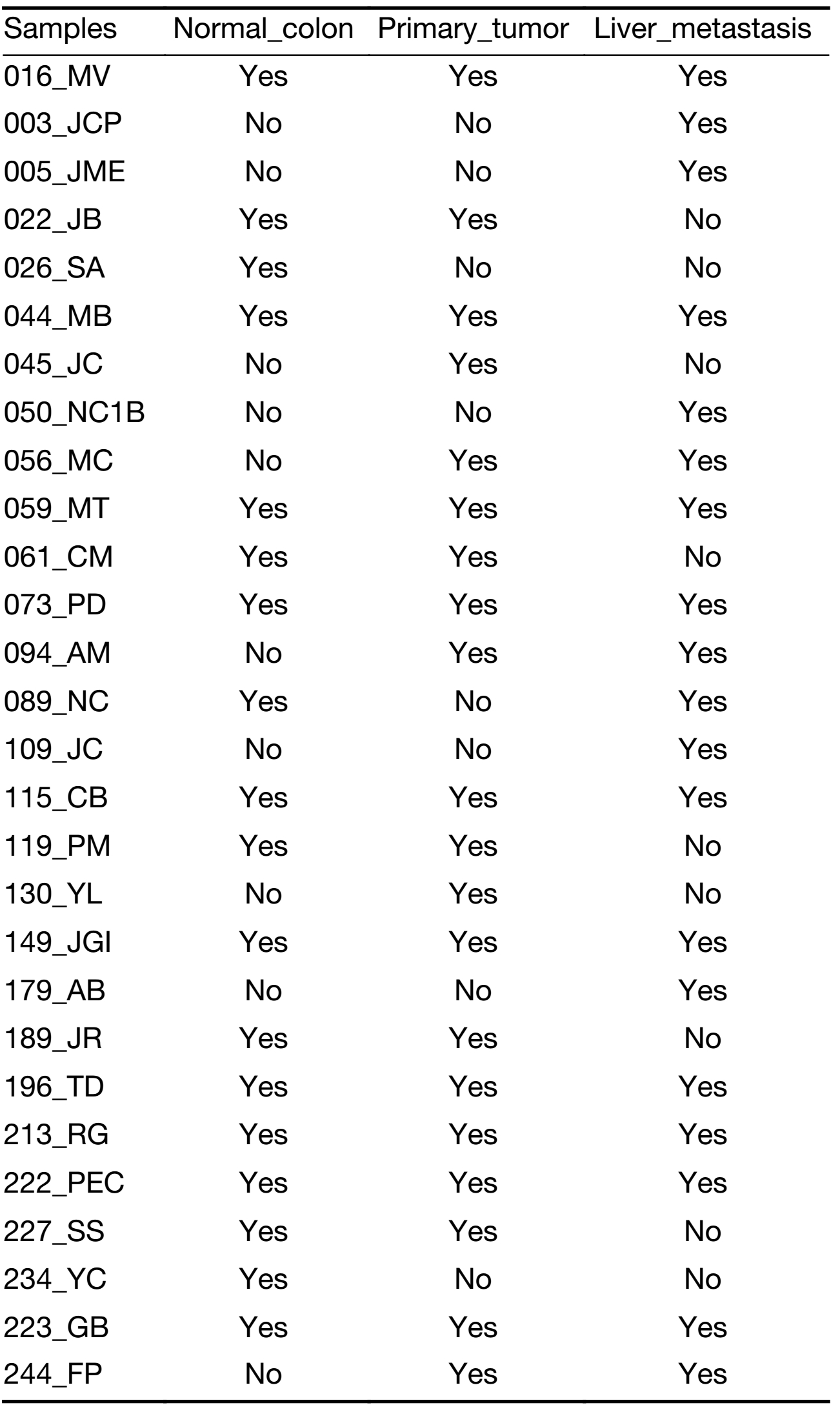
Full table
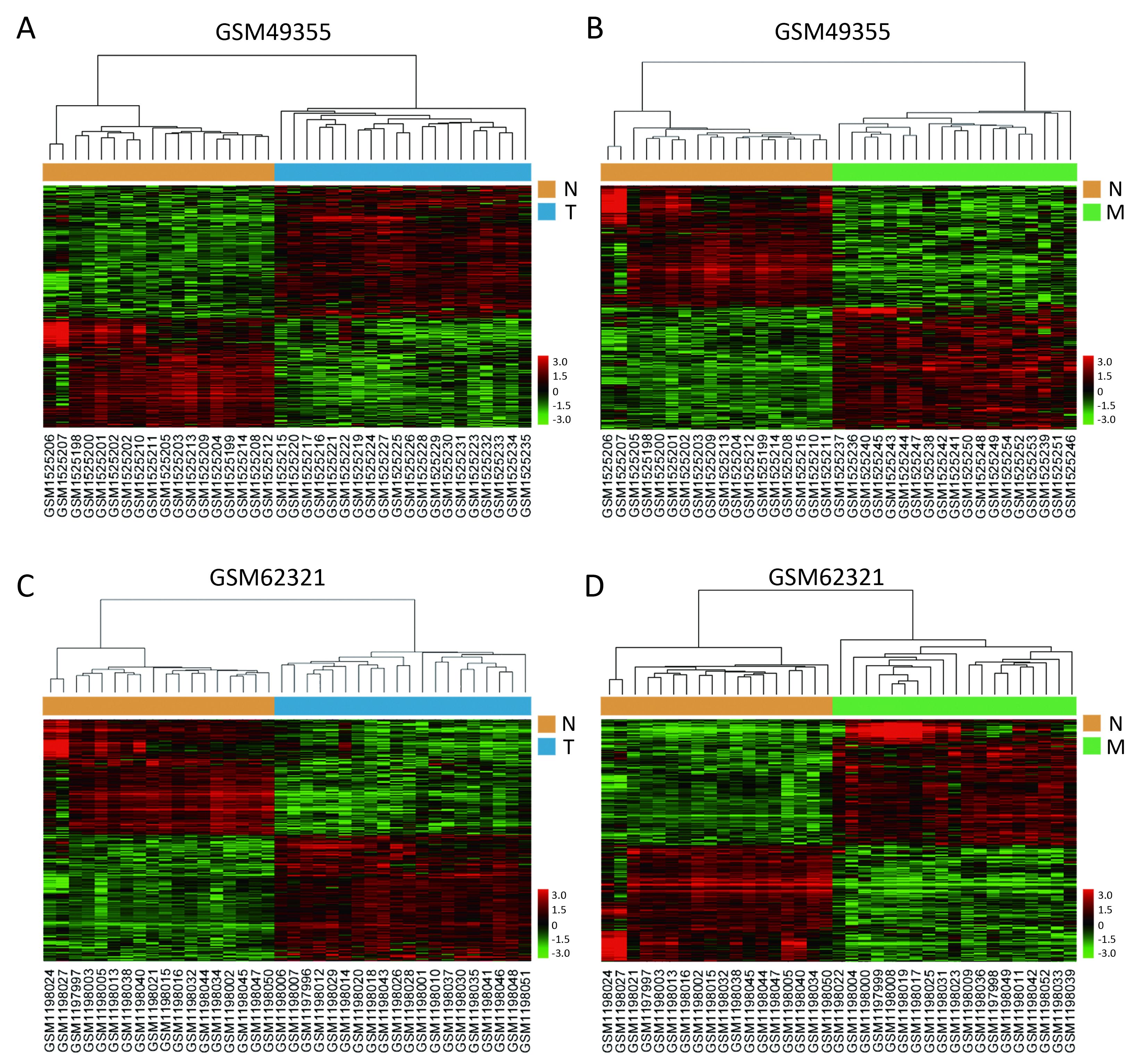
Identification of specific gene signatures
To identify molecular signatures that regulate distant metastasis in colon carcinoma, we compared mRNA expression levels in T vs. N and M vs. N. After analyzing the transcriptomic changes of T vs. N, a total of 1,646 DEGs including 861 up-regulated and 785 down-regulated transcription factors were screened out from GSE49355, and a total of 868 DEGs including 477 up-regulated and 391 down-regulated transcription factors were also identified in GSE62321. Of 1,809 DEGs, 869 were down-regulated and 940 overexpressed in M vs. N in GSE49355. The volcano plot of DEGs distribution was also presented 934 DEGs including 468 up-regulated and 466 down-regulated when comparing M with N in GSE62321 (Figure 2A, B) (P<0.05, FDR<0.05, FC>2, respectively). Based on the fact that the GSE49355 and GSE62321 were from the same panel of patients but different platform, union analysis was first performed and 719 specific genes related to liver metastasis of colon carcinoma were identified (Supplementary Table S2). However, taken into account that some of the 719 genes might be due to a single platform error, we took the intersection analysis here for obtaining higher accurate genes. The results showed that 179 genes might play an important role in the metastasis of cancer and were altered in M vs. N. Excluding 157 genes associated with tumor development, 22 genes were specific for liver metastasis (Figure 2C). Subsequently, unsupervised hierarchical cluster analysis was performed on selected 22 genes expression data using Pearson correlation-based distance and average clustering. Considerable patients’ non-pairing of N and M samples was observed in the dendrogram. Most of the specific genes showed a significantly differential expression between N and M samples (Figure 2D). Details were shown in Supplementary Table S3.
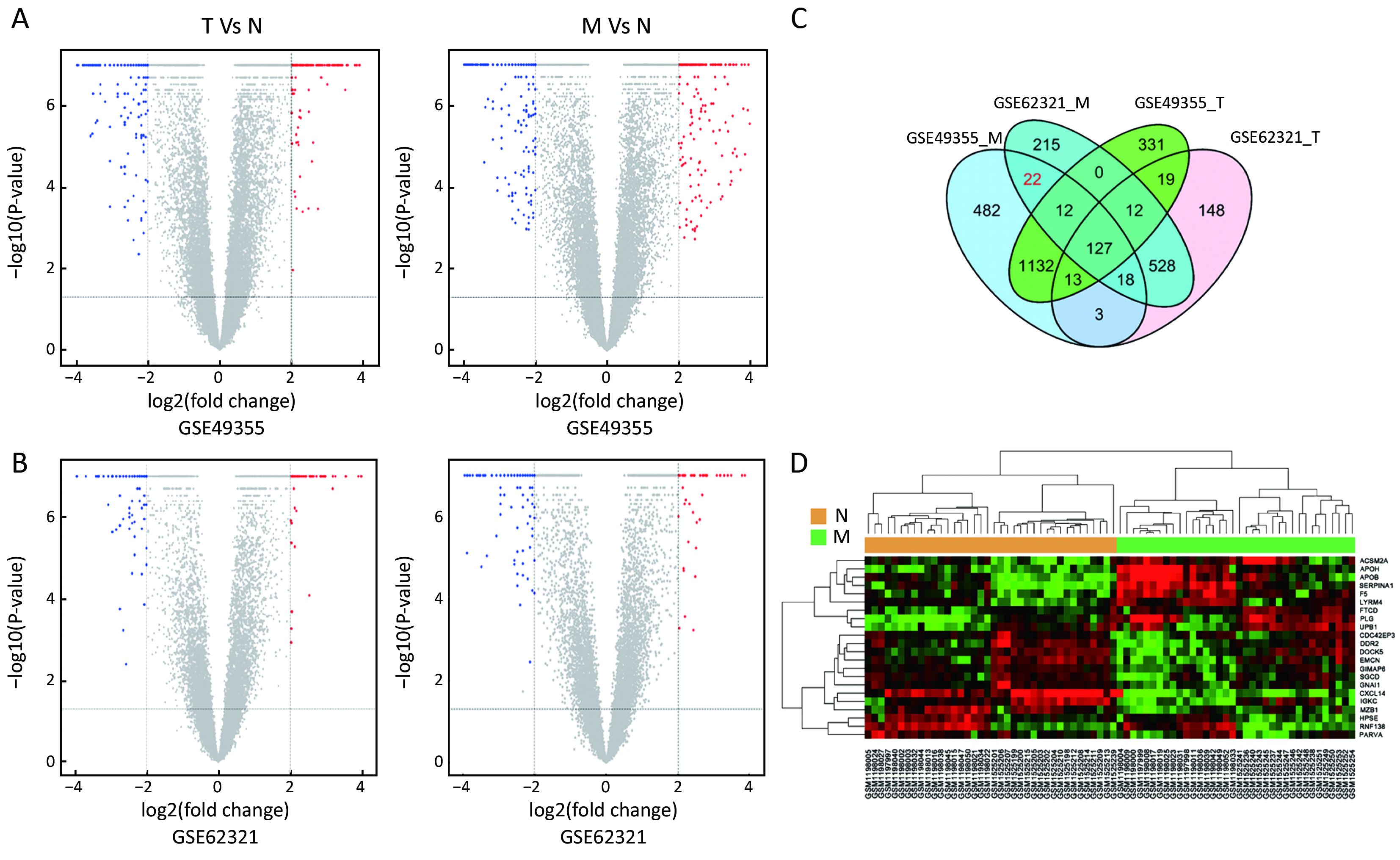
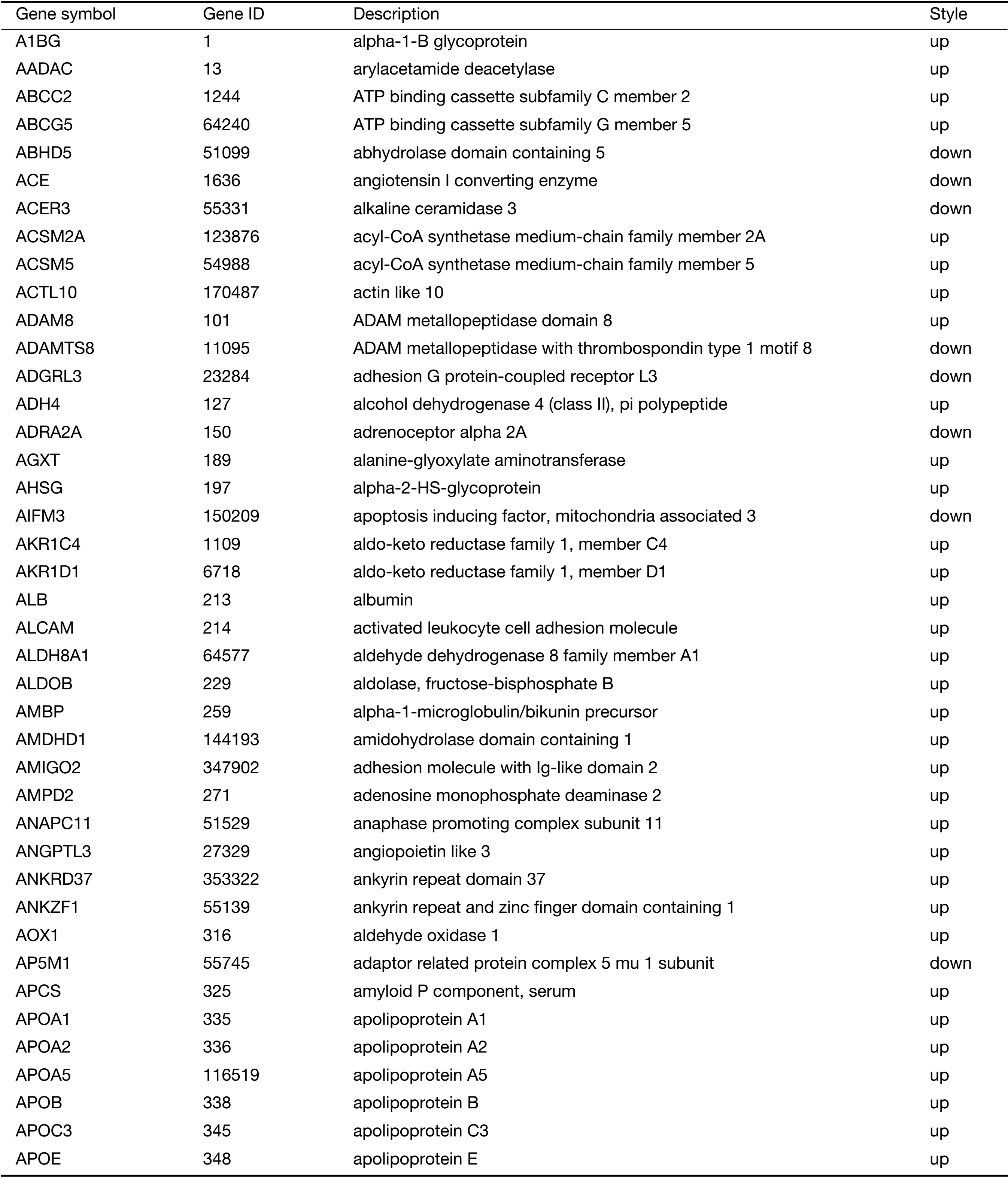
Full table
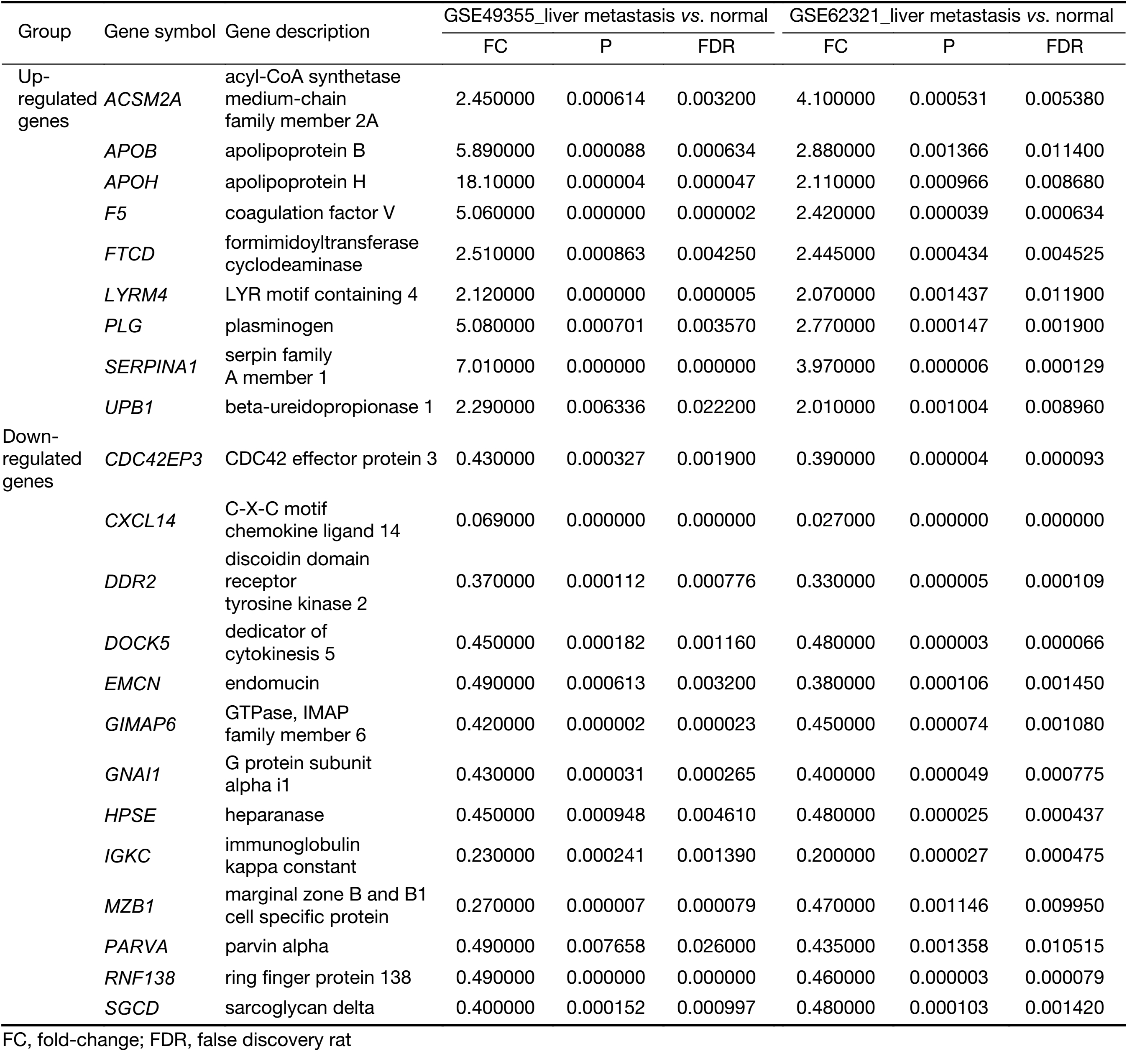
Full table
Significant GOs and pathways
All of the identified genes were used to predict the functional categories with GO annotation. They were involved in different biological processes, molecular functions and cellular components. It was found that the differential expression of the 22 genes mainly participated in 153 significant GOs (Supplementary Table S4). It was concluded that the specific genes were mainly involved in immune response, metabolic process and cell adhesion. Blood coagulation, platelet activation and degranulation, acute-phase responses, negative regulation of endopeptidase activity, and complement activation may take part in liver metastasis of colon carcinoma.
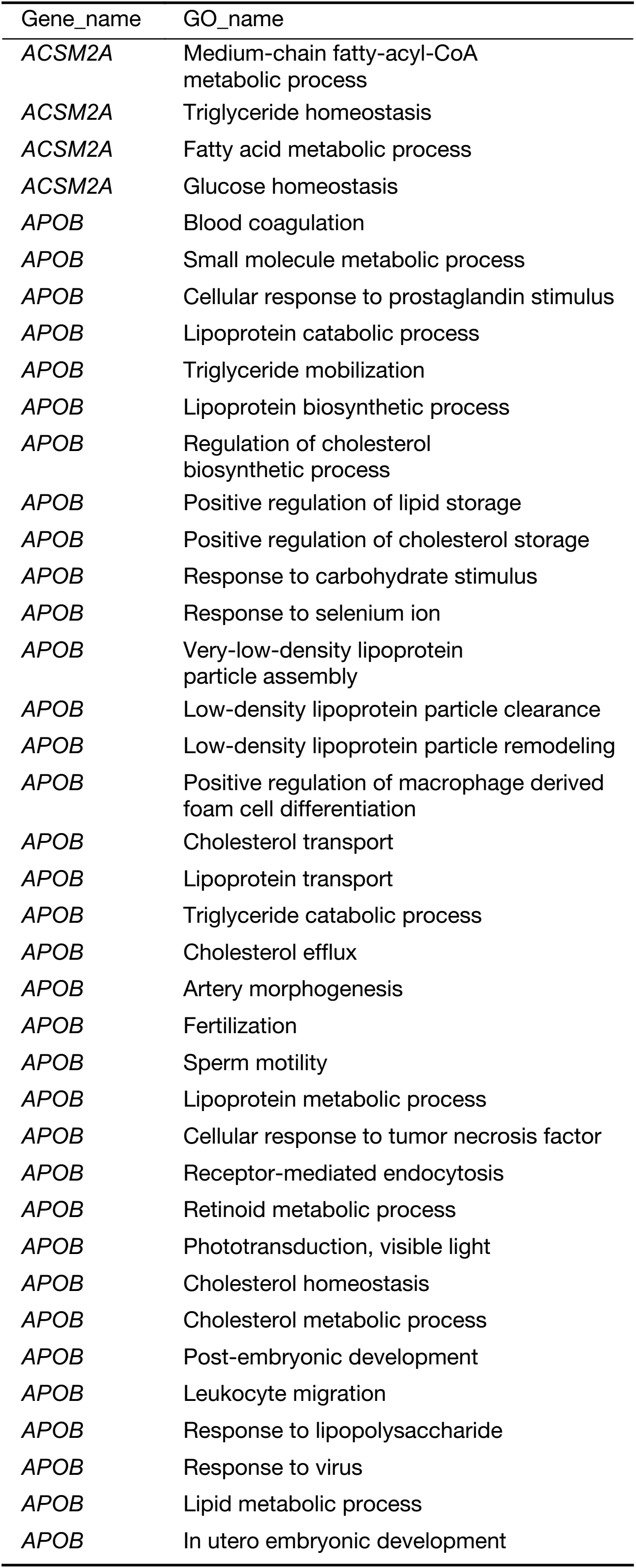
Full table
In order to identify the key pathways the specific genes were involved in, we performed pathway analysis. Fifty-six KEGG biological pathways were annotated (Supplementary Table S5). The major regulated biological pathways include complement and coagulation cascades, metabolic pathways, PI3K-protein kinase B (AKT) signaling pathway, pathways in cancer, focal adhesion, Staphylococcus aureus infection, carbon metabolism, chemokine signaling pathway, and biosynthesis of amino acids. The results revealed the genes play an important role in pathways related to cancer cell migration, such as PI3K-AKT signaling pathway, focal adhesion and chemokine signaling pathway.
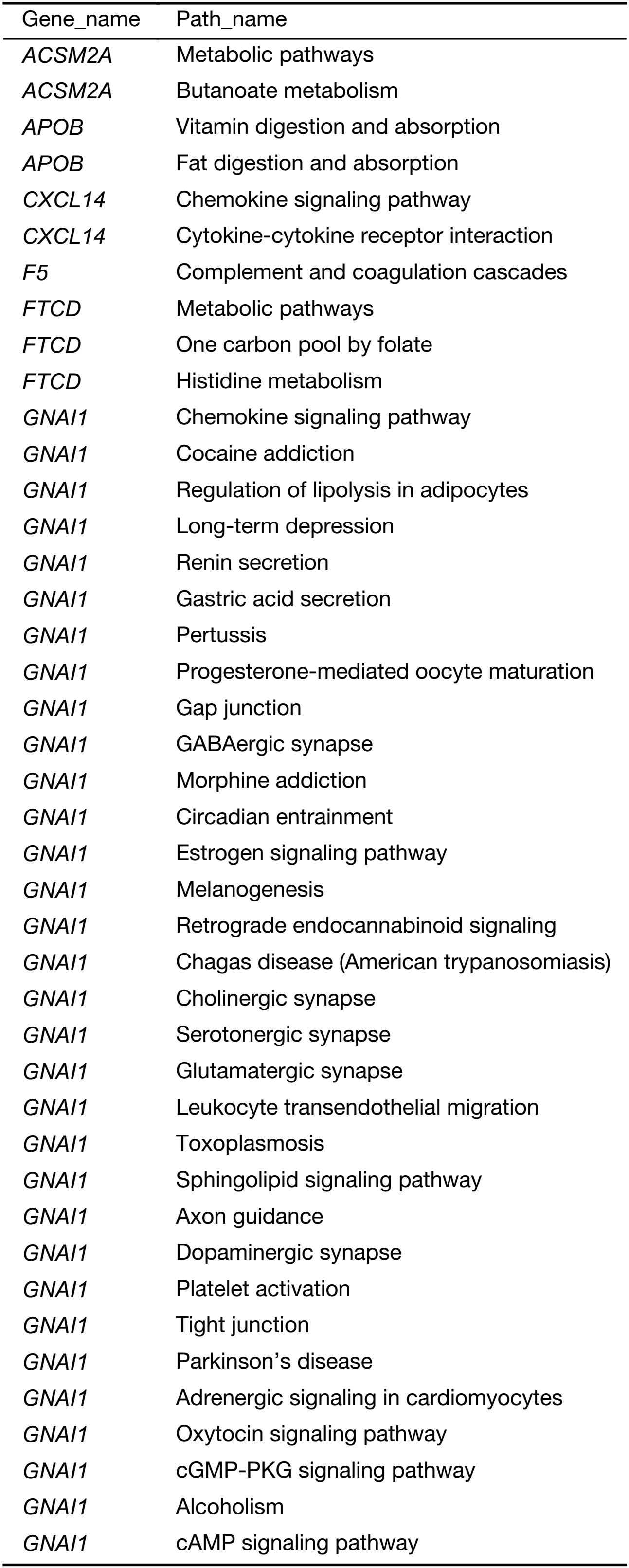
Full table
Dynamic gene network analysis
All the screened 22 DEGs were then subjected to a gene co-expression analysis network with k-core algorithm to determine which genes may play a potential role in the colon carcinoma metastasis. The gene-gene interaction network was constructed as shown in Figure 3A. The degree of a node describes the number of links of one gene with others, which had shown in the gene network. The node with larger diameter in the network means more important values. Importantly, six genes (SERPINA1, UPB1, FTCD, F5, EMCN, GIMAP6) belonged to the most significant genes, which involved in acute-phase response, metabolic process, angiogenesis, endothelial cell migration and proliferation, cell adhesion. The SERPINA1, UPB1, FTCD and F5 genes were up-regulated, but EMCN and GIMAP6 genes were down-regulated (Figure 3A). Furthermore, it was obvious that CXCL14, which was associated with cell migration and immune response, was also down-regulated.
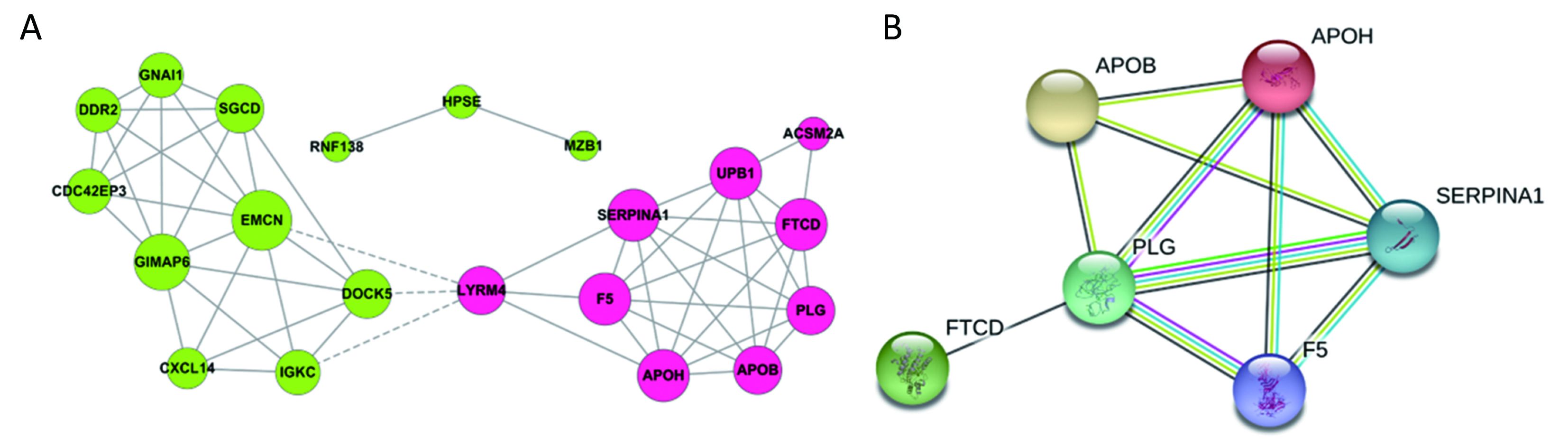
A PPI network of genes
To further define the interaction between the screened 22 DEGs, we used STRING database to construct the PPI network. The PPI network consisted of 6 nodes interacting by 29 edges, the remaining 16 DEGs failed to form the PPI pairs. It was concluded that FTCD, APOB, APOH, PLG, F5, SERPINA1 were closely linked (Figure 3B).
Prognostic values of highlighted DEGs
To evaluate the prognostic values of the 22 DEGs, we further investigated the associations of the DEGs with OS of patients by Kaplan-Meier and log-rank analysis. Because neither ACSM2A nor FTCD’s positive expression rate, the percentage of sample numbers with gene expression accounting for all sample numbers, was less than 50% in TCGA database, survival analysis was used to estimate the prognosis value of the other 20 genes. We found that patients with lower CXCL14, SERPINA1 expression demonstrated poorer survival than patients with higher expression (P=0.0388; P=0.0109; Figure 4). However, it was contradictory that SERPINA1 expression up-regulated in liver metastasis tissues indicated benefit prognosis. We therefore further researched the gene CXCL14 which were specifically involved in anti-liver metastasis process of colon carcinoma and predicted beneficial prognosis.
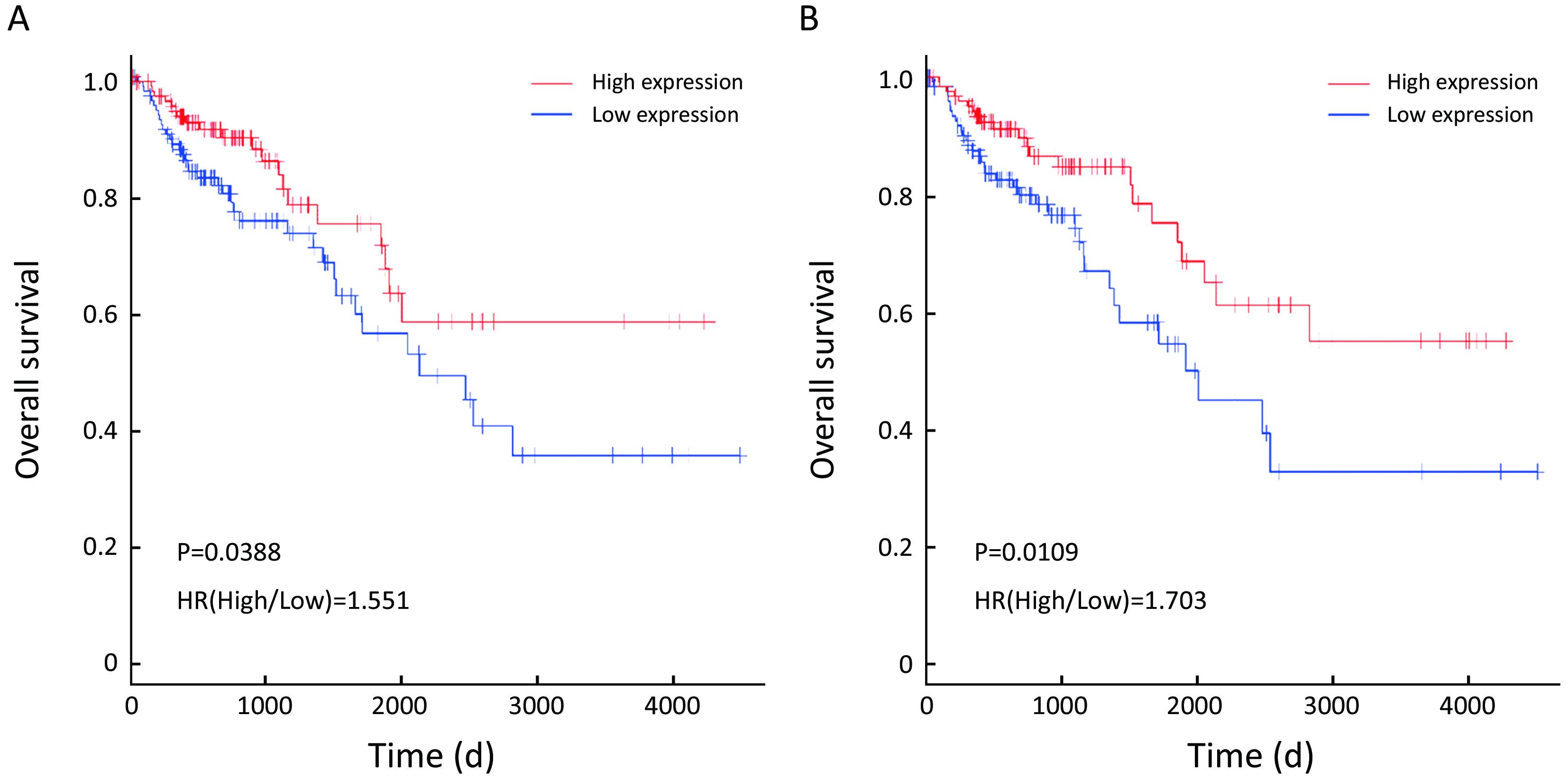
GSEA analysis of CXCL14
Based on above results, we have found that CXCL14 play a key role in liver metastasis of colon carcinoma. Then, it was quite necessary to predict biological functions of this gene. Analysis of GSEA, a powerful tool to infer the biological function, was performed. The results showed that genes associated with cell aging, negative regulation of stem cell proliferation and epithelial to mesenchymal transition (EMT), which were closely related to cancer metastasis (22-24) were significantly enriched in CXCL14-high samples of colon carcinoma (Figure 5). These observations suggested that CXCL14 may be a predicted indicator of patients with colon carcinoma liver metastasis.
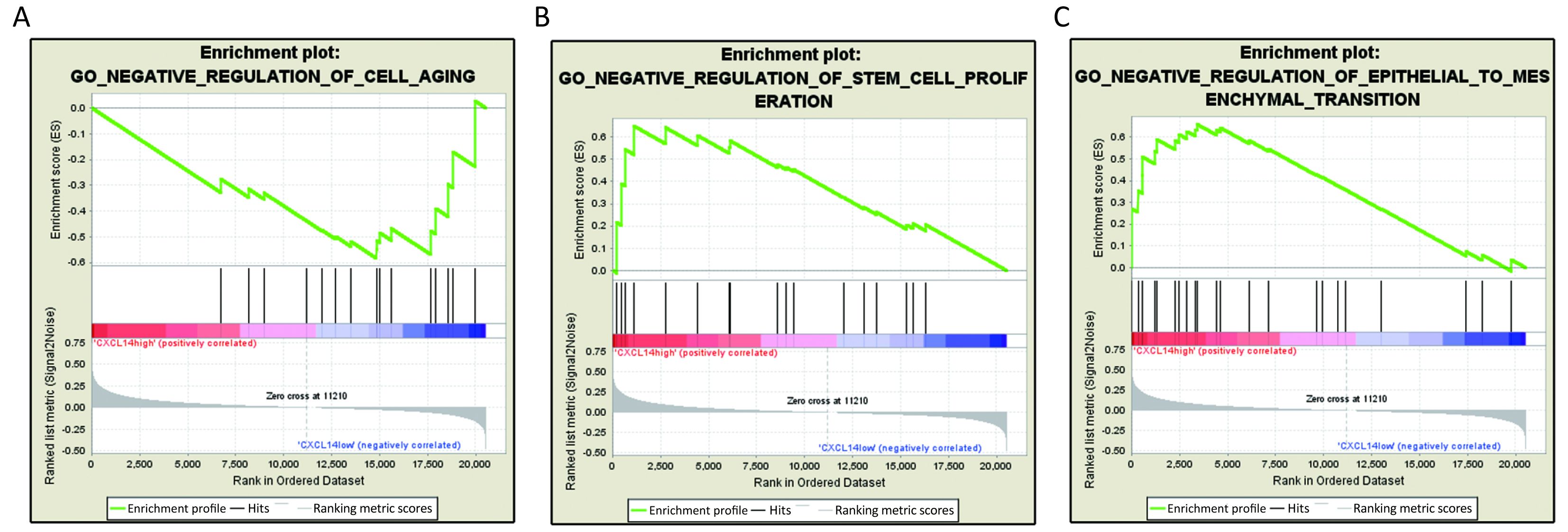
Validation of CXCL14 expression and its clinical relevance with clinical samples
To further demonstrate the clinical significance of CXCL14 expression in patients with colon carcinoma, the association between CXCL14 expression and various clinicopathological variables was investigated by real-time quantitative PCR in 103 colon carcinoma patients. The clinicopathological data of the patients are detailed in Table 1. CXCL14 expression showed a high level in colon carcinoma patients with early stage, non-liver metastasis, middle histological differentiation (Figure 6A, B, C). At the protein level, the results also showed that CXCL14 expression was lower in patients with liver metastasis (Figure 6D, E). Then 103 colon carcinoma samples were stratified into “high” and “low” according to the median 0.045 127 of CXCL14 level. We found that low expression of CXCL14 was strongly correlated with advanced liver metastasis (P=0.01), overall stage (P=0.0001), abnormal CA72-4 value (P=0.0001), tumor size (P=0.001) and site of lesion (P=0.006) (Table 2).
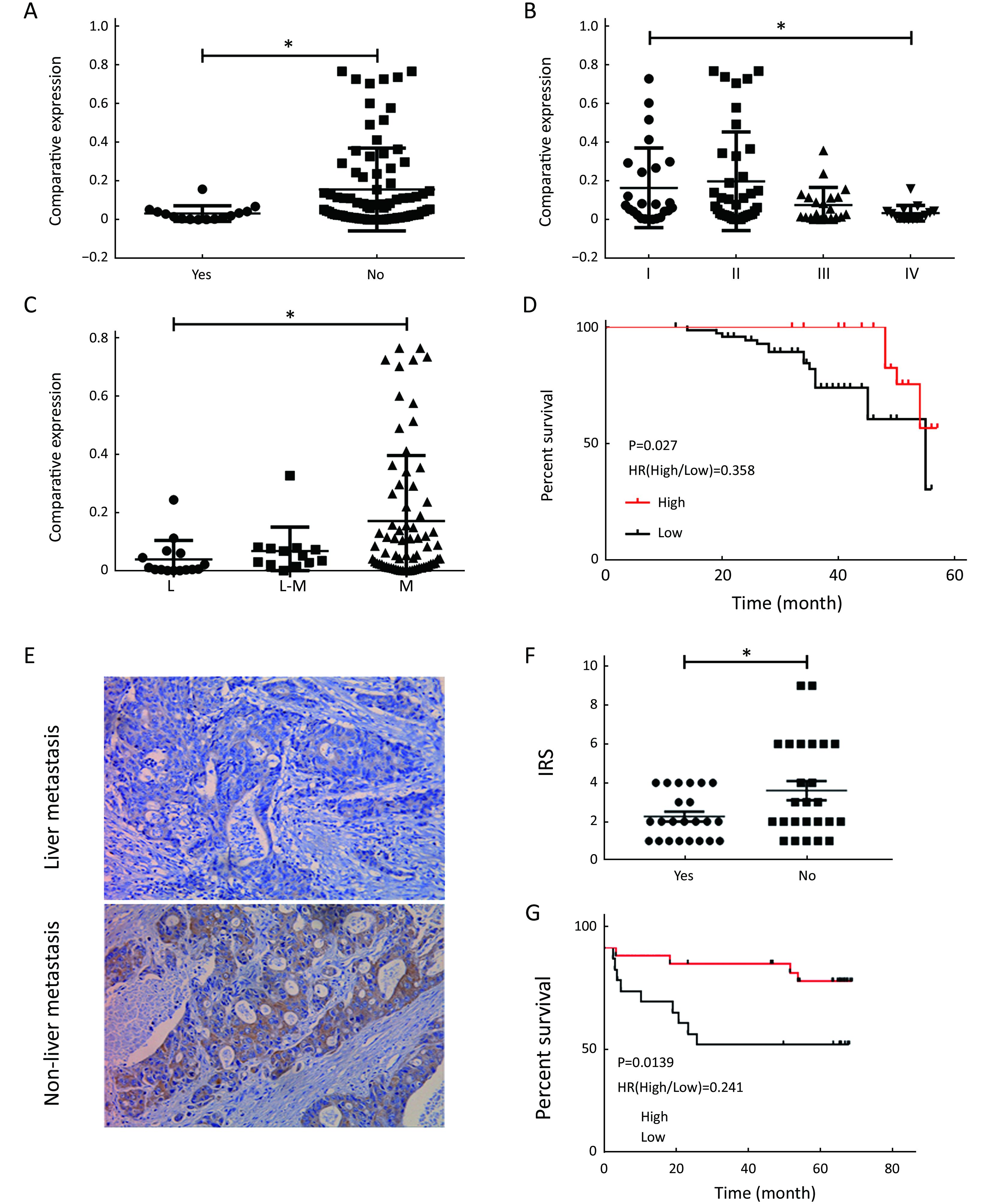
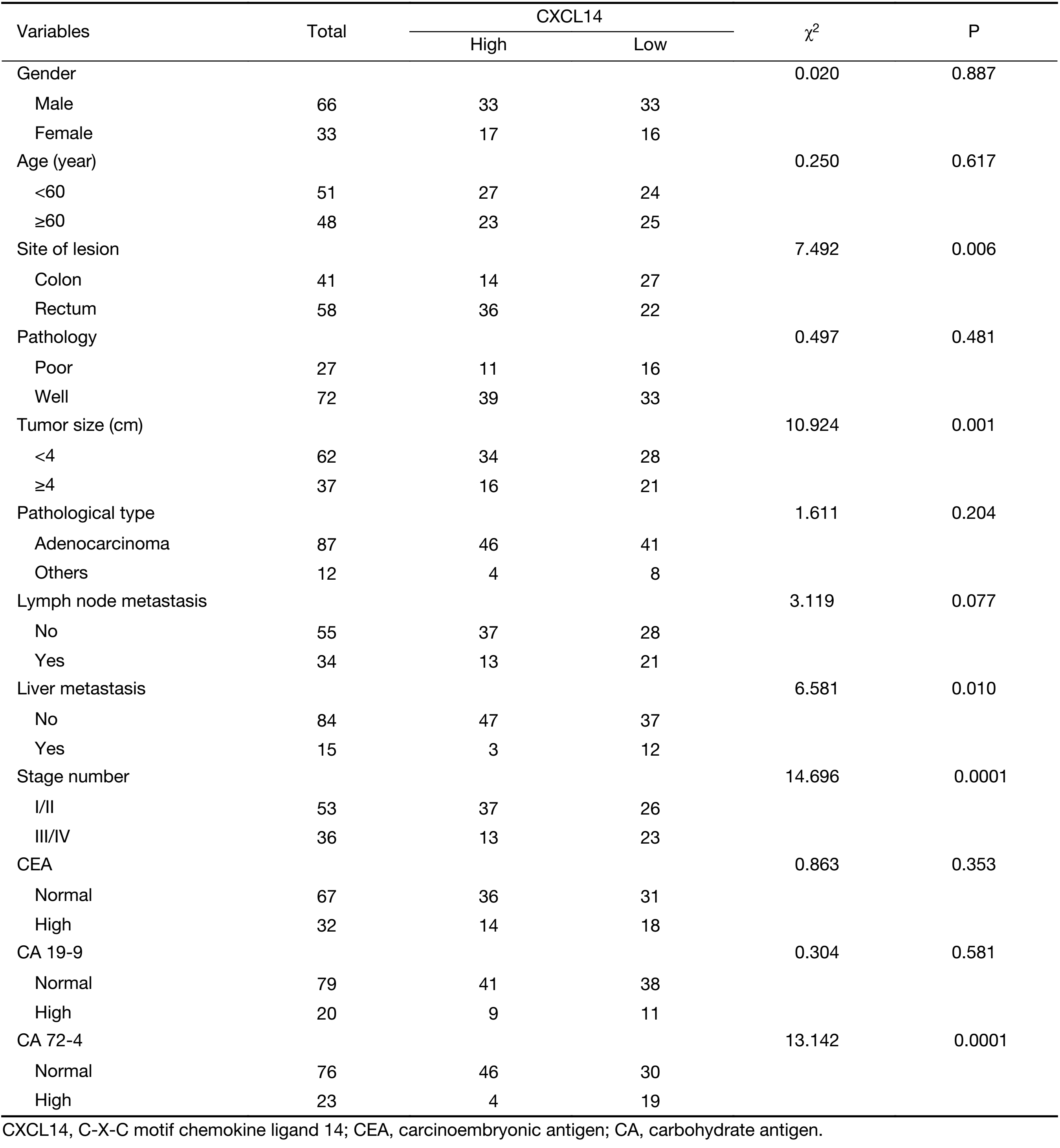
Full table
To examine the potential of CXCL14 to predict liver metastasis, logistic regression analysis was used. Univariate analyses revealed that low CXCL14 level [odds ratio (OR)=2.13; P=0.04], high CA 72-4 level (OR=6.9; P=0.01), and advanced overall stage (OR=6.0; P=0.02) were associated with liver metastasis. In multivariate analyses, CXCL14 (OR=1.24; P=0.03) and CA 72-4 levels (OR=2.35; P=0.04) were independent predictor of liver metastasis (Table 3).
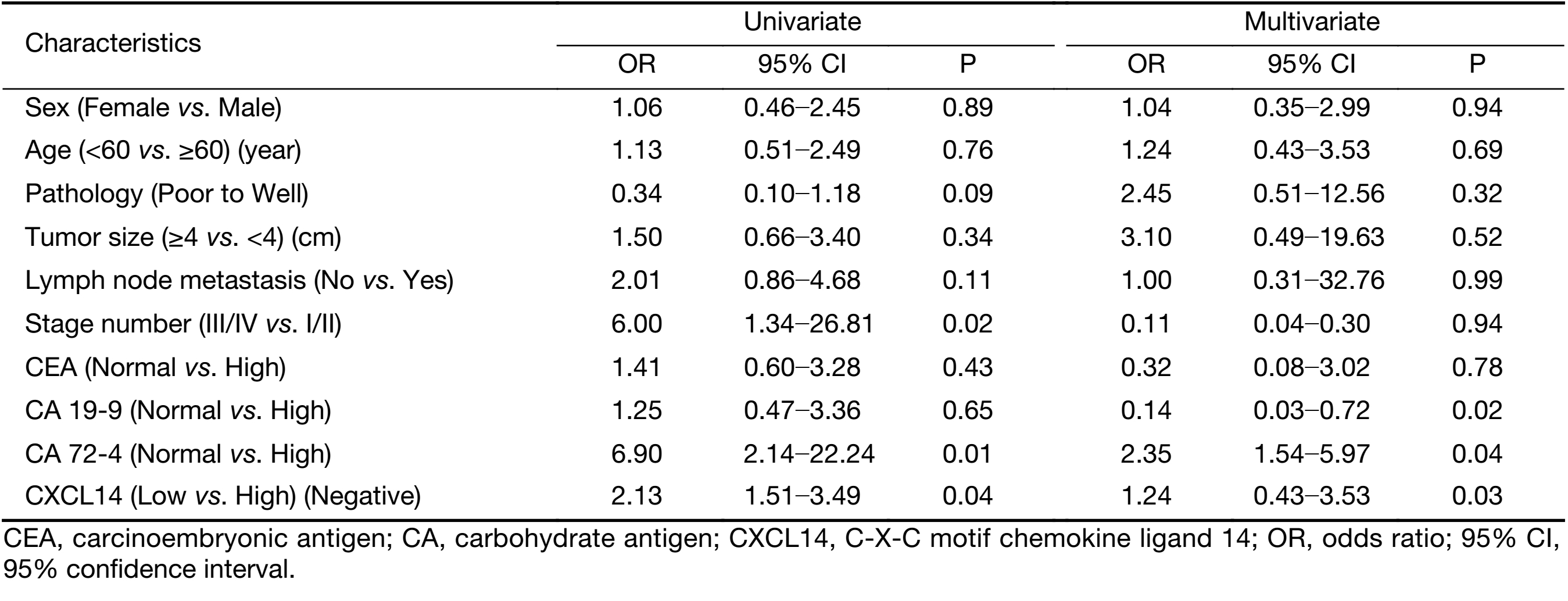
Full table
Moreover, Kaplan-Meier survival analysis revealed that higher CXCL14 expression was significantly associated with better survival in patients with colon carcinoma (Figure 6D, G). Simultaneously, as shown in Table 4, univariate analysis revealed that low CXCL14 level [hazard ratio (HR)=0.348; P=0.001] and liver metastasis (HR=2.742; P=0.037) were significantly associated with poor prognosis. Importantly, a multivariate Cox’s regression analysis revealed that CXCL14 level (HR=0.388; P=0.0001) and liver metastasis (HR=1.174; P=0.045) were independent prognostic factors for the OS of patients with colon carcinoma (Table 4). Collectively, these results suggest that CXCL14 expression status plays an important role in predicting prognosis and liver metastasis in patients with colon carcinoma.
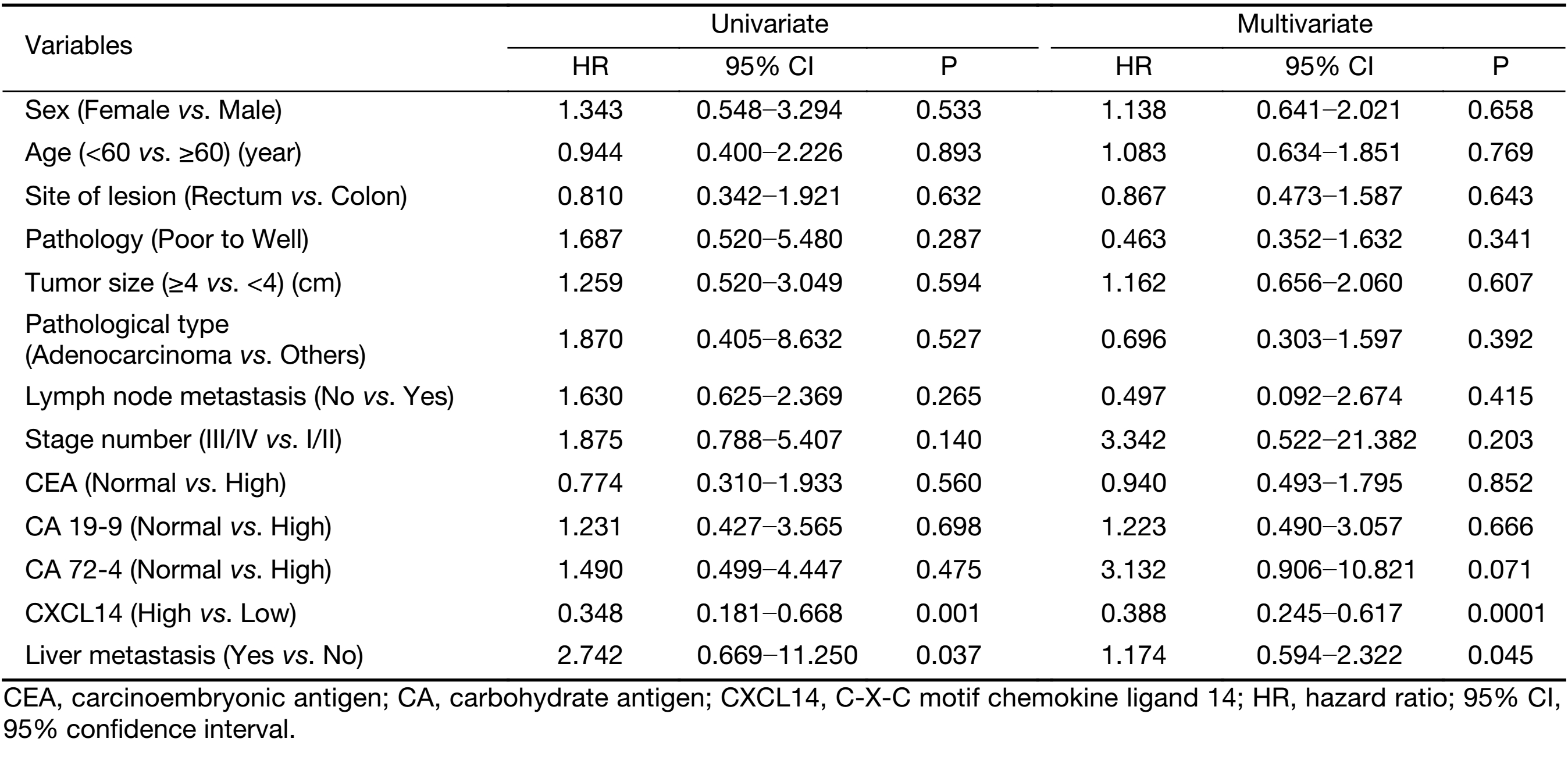
Full table
Discussion
In relation to disease relapse, liver metastasis is the most major recurrent mode of colon carcinoma. When patients were firstly diagnosed, some of them were found to have distant metastasis, which might result in unfavorable prognosis. Thus, it is critical to identify an effective indicator that predicts the liver metastasis of colon carcinoma to provide new methods for therapy. It is notable that RNA-sequencing data and microarray-based expression profiling data provide a more comprehensive and accurate understanding of carcinogenesis and cancer progression at the molecular level. In this study, mRNA profiling by microarray from GEO was used to identify a number of novel genes related to colon carcinoma liver metastasis.
To identify new predictors regulating liver metastasis in colon carcinoma, we compared mRNA expression levels in M vs. N and T vs. N. Based on the fact that the GSE49355 and GSE62321 were from the same panel of patients but different platform, we took the intersection analysis rather than the union analysis for obtaining more accurate genes. The results showed that 22 genes were specifically related to liver metastasis. To further demonstrate their function and signaling pathway, we performed annotation analysis and verified that the genes were strongly associated with: 1) cell migration, adhesion, proliferation (cell adhesion/focal adhesion/ chemokine signaling pathway/PI3K-AKT signaling pathway/APOH/F5/CXCL14); and 2) immune response (innate immune response/complement activation/acute-phase response/SERPINA1/CXCL14).
It is well known that metastasis is closely related to colon cancer patients’ survival, and almost 80% of metastases occurred in liver. Therefore, we analyzed the prognosis value of the screened 22 specific liver metastasis genes though TCGA database. Because neither ACSM2A nor FTCD’s positive expression rate was less than 50%, survival analysis was used to focus on the other 20 specific genes. The results suggest that CXCL14 and SERPINA1 may be favorable prediction factors for colon carcinoma patients’ survival. However, our present study found that SERPINA1 expressed higher level in metastatic liver tissues when comparing with normal tissues. Recent studies have been reported that SERPINA1, a protease inhibitor that can act on a variety of targets such as serine proteases, has been proposed as a poor prognosis biomarker for various diseases, including papillary thyroid carcinoma (25), lung cancer (26) and breast carcinoma (27). As for our inconsistent results of SERPINA1, we decided to focus on the CXCL14 in proceeding research.
CXCL14, is an orphan member of the CXC chemokine subfamily. CXCL14 mRNA and protein are ubiquitously expressed in normal tissues, but are absent in tumor cell lines and in primary tumors (28,29). CXCL14 level in colon carcinoma tissues with lymphoid metastasis was significantly lower than that in tumor tissues without lymphoid metastasis (30). However, the effect of CXCL14 on colon carcinoma liver metastasis remains unclear. In our study, we provided evidences to show that the expression of CXCL14 was down-regulated in M vs. N and it was closely correlated with a beneficial survival outcome. Combined with the similar results that CXCL14 mediated suppression of tumor metastasis in lung cancer and Ewing sarcoma (31), we could conclude that it may play an important role in regulating colon carcinoma metastasis. On the contrary, Liu and colleagues previously described that CXCL14 induced metastasis (32). CXCL14-positive cancer associated fibroblast involved in ovarian cancer metastatic progression. This inconsistency may be caused by the intrinsic characteristic differences of different subtypes of human cancer.
Here, we firstly reported the role of CXCL14 in colon carcinoma liver metastasis. However, its underlying mechanism remains to be elucidated. Combined with the evidence that: 1) absence of CXCL14 expression in many malignant tissues is in agreement with the deficiency of effective antitumor immune responses in cancer patients. CXCL14 may act as chemo-attractant for monocytes, dendritic cells (DC) and (natural killer) NK cells; 2) CXCL14 may also influ¬ence the proliferation, invasion and migration of tumor cells via auto/paracrine pathways; 3) CXCL14 may suppress tumor vasculature by inhibiting the chemotaxis of vascular smooth muscle cells and the formation of microvas¬cular systems (33,34), and therefore suppresses the metabolism and growth of a tumor. Thus, it might indicate that CXCL14 plays an important role in regulating liver metastasis of colon carcinoma through suppression of cancer cells and migration of leukocytes.
Our data suggest that modulating CXCL14 expression could exert tumor suppression effects on colon carcinoma. CXCL14 expression is suppressed by epidermal growth factor (EGF) and can be restored by treatment with an EGF receptor (EGFR) tyrosine kinase inhibitor in head and neck squamous cell carcinoma (HNSCC) cells (35). Reversing the promoter hypermethylation of CXCL14 could be a feasible approach to restore anti-tumor immune responses to treat oral cancers (36). In summary, up-regulating the CXCL14 level may be a valuable adjuvant treatment to improve the outcomes of patients.
Conclusions
Taken together, our data firstly indicated that the CXCL14 expression level was down-regulated in metastatic liver tissues compared to non-tumor tissues. The absence of CXCL14 contributed to the cancer metastasis that then causes poor outcomes of patients. This is the first report that CXCL14 exerts an anti-metastasis effect on colon carcinoma via the screening of bioinformatics and the further validation of clinical samples. The expression level of CXCL14 may be a valuable adjuvant parameter to predict the liver metastasis and prognosis of patients with colon carcinoma and provides a potential future therapeutic strategy.
Acknowledgements
This study was supported by grants from the National Natural Science Foundation of China (No.8177061284).
Footnote
Conflicts of Interest: The authors have no conflicts of interest to declare.
References
- Weitz J, Koch M, Debus J, et al. Colorectal cancer. Lancet 2005;365:153–65. [PubMed] DOI:10.1016/S0140-6736(05)17706-X
- Pawlik TM, Choti MA. Surgical therapy for colorectal metastases to the liver. J Gastrointest Surg 2007;11:1057–77. [PubMed] DOI:10.1007/s11605-006-0061-3
- Gao J, Li N, Dong Y, et al. miR-34a-5p suppresses colorectal cancer metastasis and predicts recurrence in patients with stage II/III colorectal cancer. Oncogene 2015;34:4142–52. [PubMed] DOI:10.1038/onc.2014.348
- Li H, Rokavec M, Jiang L, et al. Antagonistic effects of p53 and HIF1A on microRNA-34a regulation of PPP1R11 and STAT3 and hypoxia-induced epithelial to mesenchymal transition in colorectal cancer cells. Gastroenterology 2017;153:505–20. [PubMed] DOI:10.1053/j.gastro.2017.04.017
- Cohen SA, Yu M, Baker K, et al. The CpG island methylator phenotype is concordant between primary colorectal carcinoma and matched distant metastases. Clin Epigenetics 2017;9:46. [PubMed] DOI:10.1186/s13148-017-0347-1
- Galbán S, Apfelbaum AA, Espinoza C, et al. A bifunctional MAPK/PI3K antagonist for inhibition of tumor growth and metastasis. Mol Cancer Ther 2017;16:2340–50. [PubMed] DOI:10.1158/1535-7163.MCT-17-0207
- Nannini M, Pantaleo MA, Maleddu A, et al. Gene expression profiling in colorectal cancer using microarray technologies: results and perspectives. Cancer Treat Rev 2009;35:201–9. [PubMed] DOI:10.1016/j.ctrv.2008.10.006
- Kleivi K, Lind GE, Diep CB, et al. Gene expression profiles of primary colorectal carcinomas, liver metastases, and carcinomatoses. Mol Cancer 2007;6:2. [PubMed] DOI:10.1186/1476-4598-6-2
- Yamasaki M, Takemasa I, Komori T, et al. The gene expression profile represents the molecular nature of liver metastasis in colorectal cancer. Int J Oncol 2007;30:129–38. [PubMed]
- Koh KH, Rhee H, Kang HJ, et al. Differential gene expression profiles of metastases in paired primary and metastatic colorectal carcinomas. Oncology 2008;75:92–101. DOI:10.1159/000155211
- Koehler A, Bataille F, Schmid C, et al. Gene expression profiling of colorectal cancer and metastases divides tumours according to their clinicopathological stage. J Pathol 2004;204:65–74. [PubMed] DOI:10.1002/path.1606
- Del Rio M, Mollevi C, Vezzio-Vie N, et al. Specific extracellular matrix remodeling signature of colon hepatic metastases. PLoS One 2013;8:e74599. [PubMed] DOI:10.1371/journal.pone.0074599
- Lin AY, Chua MS, Choi YL, et al. Comparative profiling of primary colorectal carcinomas and liver metastases identifies LEF1 as a prognostic biomarker. PLoS One 2011;6:e16636. [PubMed] DOI:10.1371/journal.pone.0016636
- Wright GW, Simon RM. A random variance model for detection of differential gene expression in small microarray experiments. Bioinformatics 2003;19:2448–55. [PubMed] DOI:10.1093/bioinformatics/btg345
- Yang H, Crawford N, Lukes L, et al. Metastasis predictive signature profiles pre-exist in normal tissues. Clin Exp Metastasis 2005;22:593–603. DOI:10.1007/s10585-005-6244-6
- Gene Ontology Consortium. The Gene Ontology (GO) project in 2006. Nucleic Acids Res 2006;34:D322–6. [PubMed] DOI:10.1093/nar/gkj021
- Shannon P, Markiel A, Ozier O, et al. Cytoscape: a software environment for integrated models of biomolecular interaction networks. Genome Res 2003;13:2498–504. [PubMed] DOI:10.1101/gr.1239303
- Szklarczyk D, Morris JH, Cook H, et al. The STRING database in 2017: quality-controlled protein-protein association networks, made broadly accessible. Nucleic Acids Res 2017;45:D362–D368. [PubMed] DOI:10.1093/nar/gkw937
- Fischer B, Sandmann T, Horn T, et al. A map of directional genetic interactions in a metazoan cell. Elife 2015;6:4. DOI:10.7554/eLife.05464
- Liu JY, Li F, Wang LP, et al. CTL- vs Treg lymphocyte-attracting chemokines, CCL4 and CCL20, are strong reciprocal predictive markers for survival of patients with oesophageal squamous cell carcinoma . Br J Cancer 2015;113:747–55. [PubMed] DOI:10.1038/bjc.2015.290
- Guo C, Hou J, Ao S, et al. HOXC10 up-regulation promotes gastric cancer cell proliferation and metastasis through MAPK pathway. Chin J Cancer Res 2017;29:572–80. DOI:10.21147/j.issn.1000-9604.2017.06.12
- Reno TA, Kim JY, Raz DJ. Triptolide inhibits lung cancer cell migration, invasion, and metastasis. Ann Thorac Surg 2015;100:1817–24. [PubMed] DOI:10.1016/j.athoracsur.2015.05.074
- Li S, Li Q. Cancer stem cells and tumor metastasis (Review). Int J Oncol 2014;44:1806–12. [PubMed] DOI:10.3892/ijo.2014.2362
- Cai LM, Lyu XM, Luo WR, et al. EBV-miR-BART7-3p promotes the EMT and metastasis of nasopharyngeal carcinoma cells by suppressing the tumor suppressor PTEN . Oncogene 2015;34:2156–66. [PubMed] DOI:10.1038/onc.2014.341
- Vierlinger K, Mansfeld MH, Koperek O, et al. Identification of SERPINA1 as single marker for papillary thyroid carcinoma through microarray meta analysis and quantification of its discriminatory power in independent validation. BMC Med Genomics 2011;4:30. [PubMed] DOI:10.1186/1755-8794-4-30
- Topic A, Ljujic M, Nikolic A, et al. Alpha-1-antitrypsin phenotypes and neutrophil elastase gene promoter polymorphisms in lung cancer. Pathol Oncol Res 2011;17:75–80. [PubMed] DOI:10.1007/s12253-010-9283-5
- López-árias E, Aguilar-Lemarroy A, Felipe Jave-Suárez L, et al. Alpha 1-antitrypsin: a novel tumor-associated antigen identified in patients with early-stage breast cancer. Electrophoresis 2012;33:2130–7. [PubMed] DOI:10.1002/elps.201100491
- Frederick MJ, Henderson Y, Xu X, et al. In vivo expression of the novel CXC chemokine BRAK in normal and cancerous human tissue . Am J Pathol 2000;156:1937–50. [PubMed] DOI:10.1016/S0002-9440(10)65067-5
- Meuter S, Moser B. Constitutive expression of CXCL14 in healthy human and murine epithelial tissues. Cytokine 2008;44:248–55. DOI:10.1016/j.cyto.2008.08.009
- Lin K, Zou R, Lin F, et al. Expression and effect of CXCL14 in colorectal carcinoma. Mol Med Rep 2014;10:1561–8. [PubMed] DOI:10.3892/mmr.2014.2343
- Sand LG, Scotlandi K, Berghuis D, et al. CXCL14, CXCR7 expression and CXCR4 splice variant ratio associate with survival and metastases in Ewing sarcoma patients. Eur J Cancer 2015;51:2624–33. [PubMed] DOI:10.1016/j.ejca.2015.08.020
- Liu Y, Zhang J, Sun X, et al. Down-regulation of miR-29b in carcinoma associated fibroblasts promotes cell growth and metastasis of breast cancer. Oncotarget 2017;8:39559–70. [PubMed] DOI:10.18632/oncotarget.17136
- Hara T, Nakayama Y. CXCL14 and insulin action. Vitam Horm 2009;80:107–23. [PubMed] DOI:10.1016/S0083-6729(08)00605-5
- Ozawa S, Kato Y, Ito S, et al. Restoration of BRAK/CXCL14 gene expression by gefitinib is associated with antitumor efficacy of the drug in head and neck squamous cell carcinoma. Cancer Sci 2009;100:2202–9. [PubMed] DOI:10.1111/j.1349-7006.2009.01281.x
- Ang KK, Berkey BA, Tu X, et al. Impact of epidermal growth factor receptor expression on survival and pattern of relapse in patients with advanced head and neck carcinoma. Cancer Res 2002;62:7350–6. [PubMed]
- Konda JD, Olivero M, Musiani D, et al. Heat-shock protein 27(HSP27, HSPB1) is synthetic lethal to cells with oncogenic activation of MET, EGFR and BRAF. Mol Oncol 2017;11:599–611. [PubMed] DOI:10.1002/1878-0261.12042